Statistical Modeling Tools
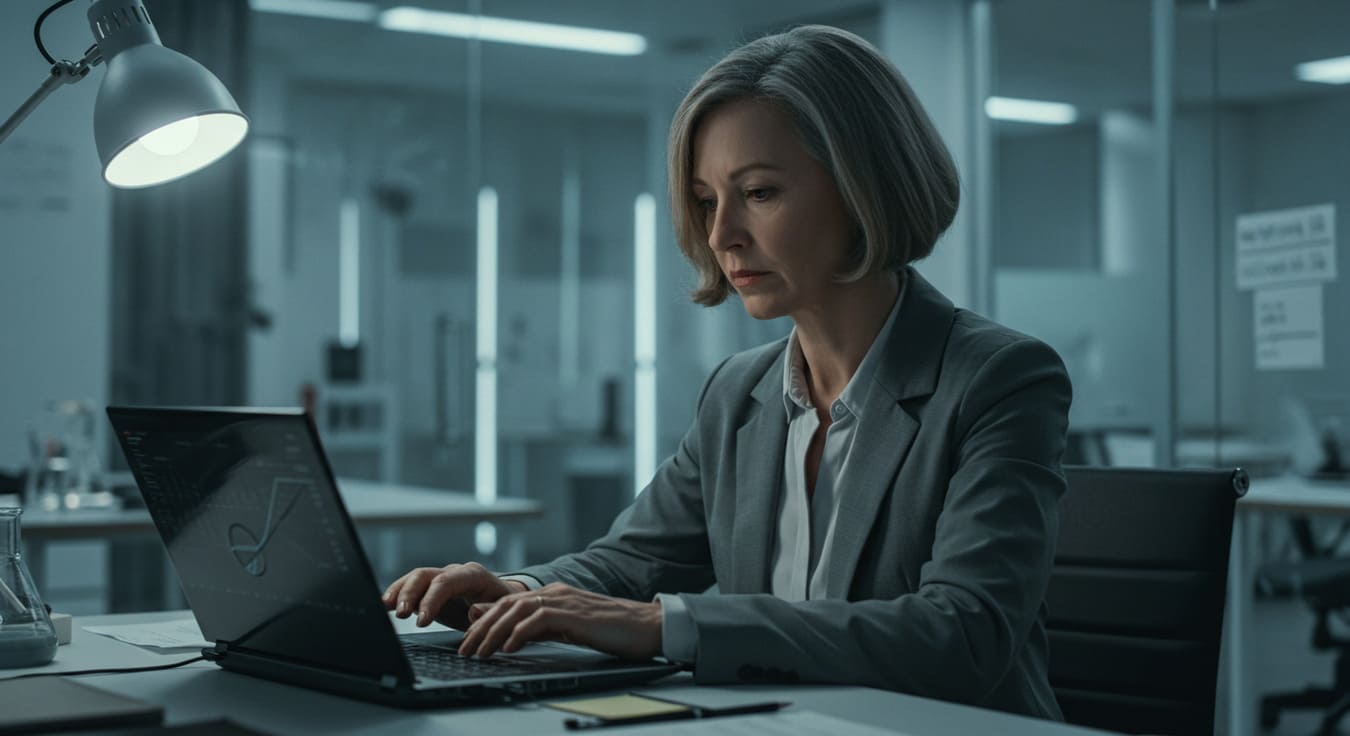
Real statistical modeling tools don’t just describe what is—they reveal what will be, why it happens, and how you can bend that future to your will.
Statistical modeling tools changed everything for businesses. They transformed data from a passive historian documenting what already happened into a crystal ball revealing what’s coming next. They replaced the expensive luxury of executive intuition with the brutal clarity of mathematical prediction.
You’re thinking… “But we already analyze our data.” Let me be brutally honest: what most companies call “analysis” is the statistical equivalent of examining a Rembrandt with a magnifying glass. You might see a few brushstrokes in excruciating detail, but you’ve completely missed the masterpiece.
Table of Contents
✅ Listen to this PODCAST EPISODE here:
The Evolution of Statistical Modeling Tools
Most executives are still trapped in analytical paradigms that would have been cutting-edge when floppy disks were revolutionary technology.
If your “advanced analytics” still center around Excel pivot tables and simplistic year-over-year comparisons, you’re bringing a spork to a gunfight in today’s competitive battlegrounds.
The journey from basic counting to sophisticated statistical modeling tools represents one of the most profound transformations in human knowledge systems—yet most business leaders remain blissfully ignorant of how far the frontier has moved beyond their understanding.
This experience highlights a crucial truth that few executives grasp: the value of statistical modeling tools comes not from their computational horsepower but from their ability to reveal causal relationships and underlying structures in your data. The most powerful tools are those that don’t just predict what will happen but explain why it happens—translating mathematical relationships into business actions.
Core Statistical Modeling Tools and Techniques That Actually Move Needles
Beneath the bewildering jargon and acronym soup of statistical modeling tools lies a surprisingly small set of techniques that consistently deliver game-changing business insights when properly applied.
Regressionsanalyse remains the workhorse of statistical modeling tools, but its implementation has evolved dramatically beyond the basic linear models most executives remember from their college statistics class. Modern regression approaches incorporate non-linear relationships, interaction effects, and hierarchical structures that capture the true complexity of business environments.
Time series forecasting has been revolutionized by ensemble methods that combine multiple statistical approaches. we worked with a specialty retailer whose inventory forecasting error rates averaged 27%—devastating for margins in a low-profit-margin business. Their traditional forecasting used simplistic moving averages that completely missed seasonal patterns and evolving trends. By implementing a hybrid forecasting model that combined ARIMA structures, exponential smoothing, and machine learning algorithms with external variables like weather patterns and local economic indicators, we slashed forecast error to just 6.8%. This improvement decreased inventory costs by $7.3M annually while simultaneously reducing stockouts by 71%.
Propensity modeling has transformed marketing effectiveness by identifying which customers are most likely to respond to specific initiatives. The statistical sophistication here isn’t just about predicting response rates—it’s about calculating the incremental impact of marketing interventions.
Structural equation modeling represents one of the most powerful yet underutilized statistical approaches in business. Unlike simpler techniques, these models can simultaneously test multiple causal relationships between observed and latent variables. For a subscription software company plagued by churn, this approach revealed that product usage affected retention through three separate pathways with different time horizons: directly through immediate value creation, indirectly through habit formation, and through network effects that increased switching costs. This nuanced understanding allowed them to redesign their onboarding and engagement strategies around these specific causal pathways, reducing churn by 24% within two quarters.
Bayesian analysis has emerged as particularly valuable for decisions under uncertainty—which describes virtually all significant business decisions. Unlike traditional statistical approaches that generate single-point estimates, Bayesian methods produce full probability distributions that quantify uncertainty.
From Data to Decisions: Implementing Models That Actually Matter
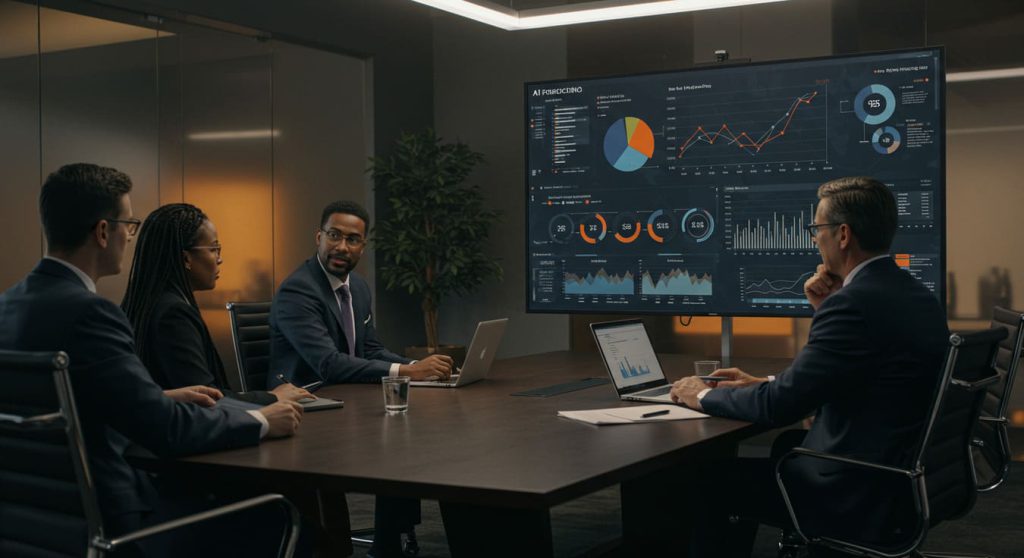
After guiding hundreds of organizations through this treacherous terrain, these implementation approaches consistently succeed:
Start with business problems that actually matter, not techniques that sound impressive. Countless initiatives crash and burn because they began with a solution (“We need to implement machine learning!”) rather than a problem (“Why are we losing our highest-value customers?”). Successful implementations always begin with specific, consequential business questions that, if answered, would directly impact decisions worth millions.
Data preparation is where battles are won or lost, yet it’s the part everyone wants to skip. Here’s the unsexy truth about statistical modeling tools: 60-80% of the work happens before any actual modeling begins.
Model development should be iterative, transparent, and pragmatic. The black box approach to statistical modeling creates models that executives don’t trust and can’t explain—ensuring they’ll be ignored when it really matters. When we developed market response models for a consumer packaged goods company, we followed a deliberately transparent process: starting with simpler models whose results were intuitive and credible, gradually adding complexity while validating that each addition meaningfully improved predictive power, and creating clear visualizations showing how input factors influenced the predictions.
Validation must be brutally rigorous and business-relevant. Many companies bet millions on models with impressive-looking statistical metrics that proved completely worthless in practice.
Implementation requires translation, not just calculation. The most brilliant statistical model creates precisely zero value if its insights aren’t implemented. For every modeling project, we create decision support tools that translate statistical outputs into specific business actions.
Emerging Frontiers: Where Statistical Modeling Is Heading
Causal machine learning represents the holy grail that’s finally coming into reach. Traditional machine learning excels at finding patterns and making predictions but falls flat on its face when asked about causality—the critical “why” behind outcomes that actually drives decisions. New approaches combining econometric techniques with machine learning are creating models that don’t just predict but explain.
Automated model generation and selection is slashing the time required to develop robust models from months to hours. Systems that can automatically test thousands of model specifications and select optimal approaches based on predictive accuracy and business constraints are democratizing capabilities that once required Ph.D. statisticians. However, this automation creates both opportunity and peril.
Transfer learning techniques are allowing companies to leverage insights across seemingly unrelated domains. Rather than building each statistical model from scratch, these approaches adapt existing models from other contexts, dramatically accelerating development.
Integrated decision optimization represents perhaps the most transformative frontier, connecting statistical modeling directly to automated decision systems. These approaches don’t just predict outcomes—they recommend optimal actions to achieve business objectives under constraints.
The Human Element: Building Statistical Literacy That Sticks
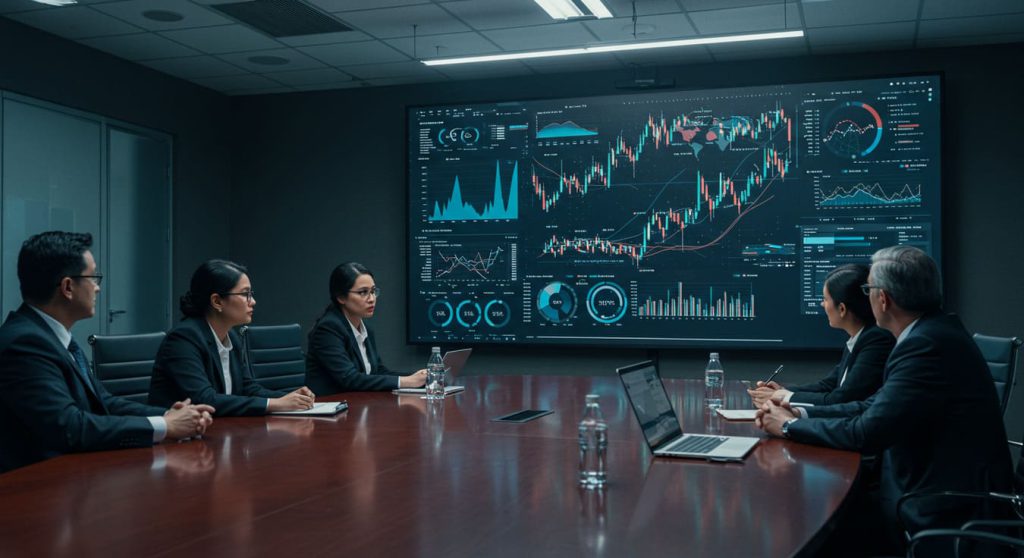
The uncomfortable truth about statistical modeling tools is that their effectiveness depends as much on the humans using them as on the mathematics behind them. You can implement the most sophisticated models available, but if your organization lacks the statistical literacy to properly interpret and apply them, you’re building analytical castles on quicksand.
This human dimension of statistical modeling is frequently overlooked, yet it’s often the determining factor between organizations that extract transformative value from these tools and those that achieve nothing beyond impressive-sounding technical capabilities and fancy dashboards no one uses. After guiding hundreds of companies through this journey, I’ve identified the approaches that consistently build the necessary human capabilities alongside the technical ones.
Start by demystifying statistics through business relevance. One of the biggest barriers to effective use of statistical modeling tools is the intimidation factor—the perception that statistics requires mathematical genius to understand. Recognize that statistical literacy isn’t one-size-fits-all. Different roles need different types and depths of understanding. Executives need enough literacy to understand the strategic implications of model outputs and their limitations without getting lost in technical details. Analysts need deeper knowledge to properly specify and interpret models. Operational staff need practical understanding of how model outputs should influence their daily decisions.
Build institutional memory for statistical learning. One of the most insidious challenges in building statistical literacy is knowledge attrition—insights and lessons learned disappear as people change roles or leave the organization. To combat this, you can create knowledge repositories that document not just models but the reasoning behind methodological choices, lessons learned from failed approaches, and the evolution of analytical understanding over time.
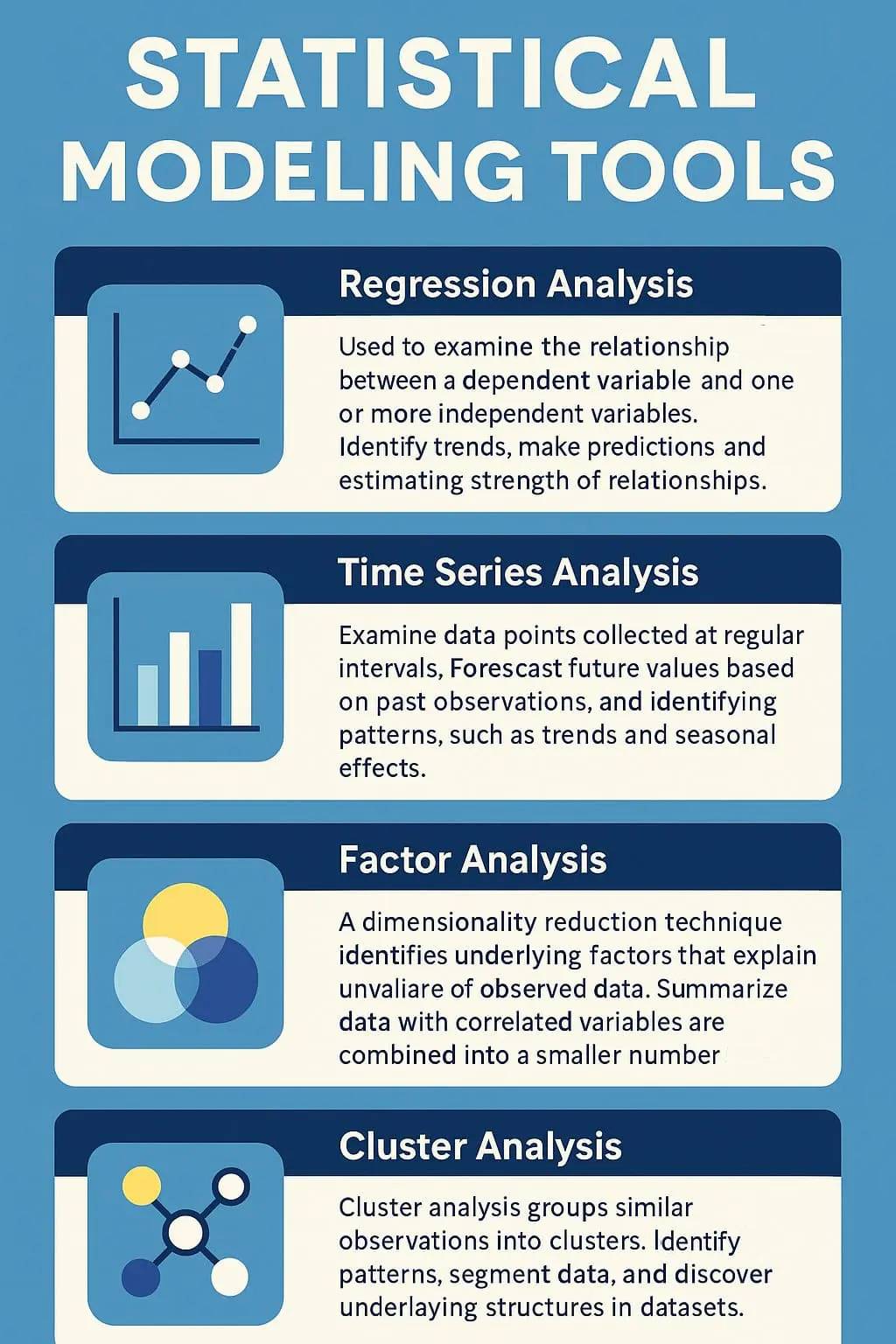
Key Takeaways: Statistical Modeling Tools
✅ Statistical modeling tools have evolved from simplistic descriptive approaches to sophisticated predictive and prescriptive capabilities that transform raw data into strategic foresight—but most companies remain trapped in analytical paradigms that would have been cutting-edge when fax machines were revolutionary
✅ The most powerful statistical approaches don’t just predict outcomes but reveal causal relationships and underlying structures that explain why events occur and how to influence them—moving beyond correlation to the causation that actually drives effective business decisions
✅ Successful implementation requires more than technical sophistication—it demands clear business problems worth solving, rigorous data preparation, transparent model development, and seamless integration with existing decision processes
✅ Emerging capabilities including causal machine learning, automated modeling, and integrated decision optimization are redefining what’s possible in business analytics, creating both extraordinary opportunities and existential threats for unprepared organizations
✅ Common pitfalls include the fetishization of unnecessary complexity, data leakage creating false confidence, overfit models that fail in practice, mistaking correlation for causation, and siloed implementation that never impacts decisions
✅ Building statistical literacy throughout the organization is as important as implementing the technical tools—without human understanding, even the most sophisticated models create limited value and often gather dust while critical decisions continue to be made based on intuition and flawed analysis
What Makes SIS International a Top Statistical Modeling Partner?
When selecting a partner for implementing statistical modeling tools, methodological expertise alone isn’t sufficient—you need a team that bridges the critical gap between analytical sophistication and actual business impact. After four decades pioneering these approaches across industries and watching countless implementations succeed or fail, here’s what truly differentiates our capabilities:
✔ GLOBAL REACH: Statistical relationships vary dramatically across markets due to cultural, economic, and competitive differences that generic models completely miss. Our presence in 120+ countries allows us to develop models that capture these critical variations rather than applying one-size-fits-all approaches that inevitably fail.
✔ 40+ YEARS OF EXPERIENCE: We’ve evolved with statistical methodology from the mainframe era through today’s AI-enhanced modeling. This historical perspective means we can select the right analytical approach for each business question rather than being limited to whatever technique happens to be fashionable in academic journals.
✔ GLOBAL DATA BASES FOR RECRUITMENT: The strongest statistical models require both internal company data and external market information that most organizations simply don’t have. Our proprietary respondent databases provide unique access to targeted consumer and B2B populations, allowing us to gather the precise data needed to build comprehensive market models.
✔ IN-COUNTRY STAFF WITH OVER 33 LANGUAGES: Effective statistical modeling requires deep understanding of context, including cultural and linguistic nuances that affect market behavior in ways that aren’t captured in raw data.
✔ GLOBAL DATA ANALYTICS: Our dedicated analytics teams specialize in the full spectrum of statistical modeling approaches, from traditional econometrics to cutting-edge machine learning techniques. This breadth of expertise means we select methodologies based on their appropriateness for your specific business questions rather than forcing your needs to fit our capabilities—a common problem with firms that specialize in only one analytical approach and try to apply it universally.
✔ AFFORDABLE RESEARCH: Our global scale and methodological efficiency allow us to deliver sophisticated statistical modeling at price points significantly below typical management consulting rates. We’ve structured our approach to deliver maximum insight value without the bloated timelines and teams that drive up costs elsewhere.
✔ CUSTOMIZED APPROACH: We don’t believe in one-size-fits-all methodologies. Each statistical modeling project is tailored to your specific business questions, data environment, and implementation context.
FAQs: Statistical Modeling Tools
What exactly are statistical modeling tools and how do they differ from basic analytics?
Statistical modeling tools are methods and software that go beyond showing what happened to revealing why it happened and what will happen next under different conditions. While basic analytics might tell you that sales dropped 15% in a region (the what), proper statistical modeling would identify which specific factors caused that decline, how those factors interacted in non-linear ways, and how similar conditions might affect other regions in the future (the why and what next). The fundamental difference is that basic analytics summarizes historical data points, while statistical modeling extracts the underlying patterns and relationships that generate those data points.
Which statistical modeling approaches deliver the most business value in real-world applications?
After implementing hundreds of modeling initiatives across virtually every industry, we’ve found that certain approaches consistently deliver exceptional ROI when properly applied to the right business problems. Multivariate regression techniques remain workhorses because they explicitly quantify the relationship between multiple input factors and business outcomes while controlling for confounding variables—and they do so in ways business leaders can actually understand.
Time series modeling delivers particular value for any business facing cyclical patterns or trends that simple year-over-year comparisons completely miss. Market response modeling that quantifies how business outcomes respond to controllable factors like pricing, promotion, and product features typically yields immediate ROI by optimizing resource allocation across competing priorities. Structural equation modeling excels for complex customer behaviors where multiple factors interact through various pathways rather than in simple linear relationships.
Unser Standort in New York
11 E 22nd Street, Floor 2, New York, NY 10010 T: +1(212) 505-6805
Über SIS International
SIS International bietet quantitative, qualitative und strategische Forschung an. Wir liefern Daten, Tools, Strategien, Berichte und Erkenntnisse zur Entscheidungsfindung. Wir führen auch Interviews, Umfragen, Fokusgruppen und andere Methoden und Ansätze der Marktforschung durch. Kontakt für Ihr nächstes Marktforschungsprojekt.