K-Means Cluster Analysis in Market Research
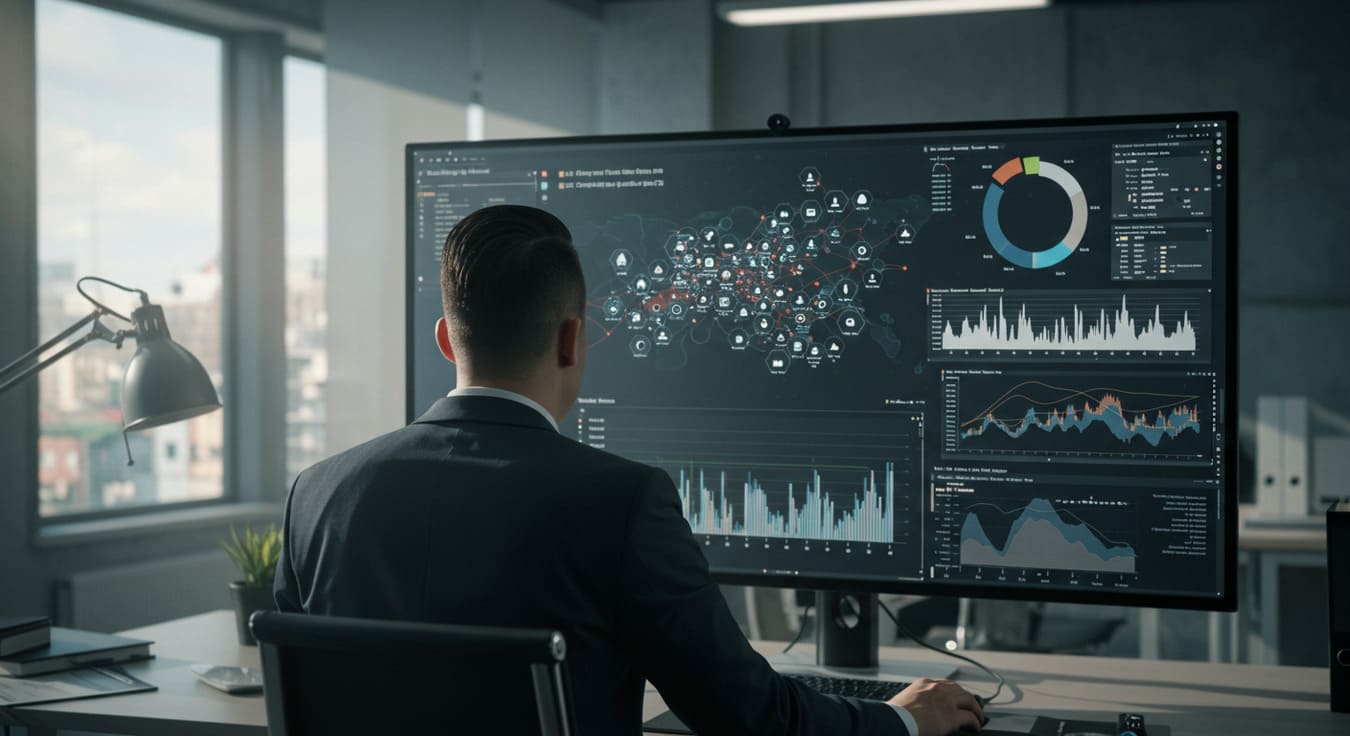
The companies thriving today are using K-means to see patterns their competitors can’t—moving with surgical precision while others flail with sledgehammers.
Ever wondered how companies like Apple or Netflix seem to know exactly what you want before you do? The secret sauce isn’t mind reading—it’s advanced data analysis. K-means cluster analysis in market research has transformed how we understand customer behavior.
Let me show you why this matters more than ever in our data-saturated world—and why most companies are still getting it catastrophically wrong.
Table of Contents
✅ Listen to this PODCAST EPISODE here:
What is K-Means Cluster Analysis in Market Research?
K-means cluster analysis in market research is like letting your customers organize themselves naturally rather than jamming them into arbitrary buckets labeled “millennial” or “suburban dad.” The algorithm finds patterns in behavior, preferences, and needs—grouping similar customers based on what they actually do instead of who you think they are.
The “K” is simply the number of clusters you’re looking for. Too few, and you miss critical distinctions. Too many, and you’re chasing ghosts.
Back in the early 2000s, mentioning K-means cluster analysis in market research got you blank stares or eye-rolls from most executives. “Just give me demographics!” they’d say. But, demographics tell you superficial facts. Clustering reveals the psychological and behavioral truths driving purchase decisions.
The Strategic Benefits of K-Means Cluster Analysis
Why has K-means become my non-negotiable recommendation for clients facing market complexity? The strategic advantages transcend mere “better segmentation.”
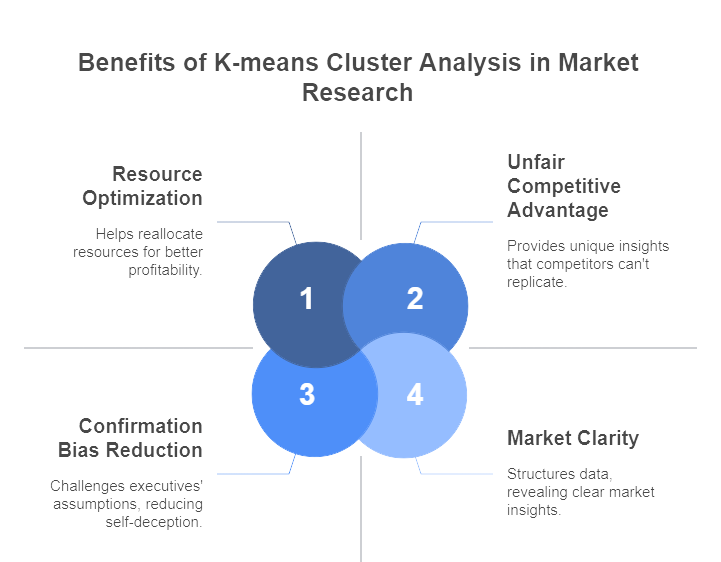
First, it murders confirmation bias. Most traditional research just confirms what executives already think they know. It’s expensive, comforting self-deception. K-means cluster analysis in market research regularly produces findings that make executives physically uncomfortable—because it challenges their core assumptions about their market.
One hospitality CEO actually got angry when clustering revealed their highest-value customer segment wasn’t the demographic they’d built their entire brand around. Six months later, he sent me a handwritten thank-you note. Their stock was up 26%.
Second, it creates unfair competitive advantage. Anyone can buy demographic data. The insights from sophisticated clustering are unique to your customer base and market position. Competitors can’t replicate your K-means findings because they don’t have access to your unique customer behavior data.
Third, it stops resource hemorrhaging. A consumer packaged goods client discovered through K-means analysis that they were overspending on their largest customer segment (who were price-sensitive bargain hunters) while practically ignoring a smaller but fanatically loyal segment. Reallocating just 15% of their marketing budget increased overall profitability by 11%.
Finalmente, it creates clarity in a world drowning in data. The problem isn’t lack of information—it’s lack of structure in that information. K-means cluster analysis in market research is like suddenly finding the constellations in a sky full of random stars.
Choose Your Variables Like Your Life Depends On It
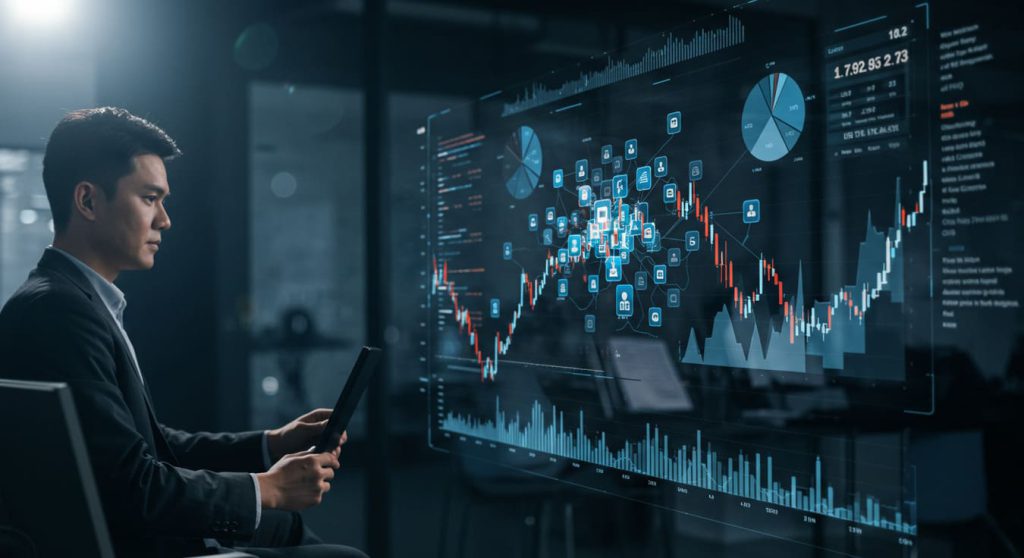
The best variables tell stories. The worst just tick boxes.
Don’t just grab convenient demographic data. Focus relentlessly on behaviors and attitudes that actually drive purchasing decisions.
Successful projects typically work with 15-30 key variables representing customer behaviors, preferences, and pain points. A financial services project transformed when they moved beyond basics like account balance to include engagement patterns, support interactions, and life event indicators.
Stop Asking “How Many Clusters Should We Use?”
This question misses the point entirely. While there are statistical methods like the elbow method or silhouette analysis, the truth is that the best number balances statistical validity with business usability.
Start with a range of cluster solutions (typically 3-8) and evaluate each not just on mathematical fit, but on whether the resulting segments tell a coherent story that can drive action. Sometimes a 5-cluster solution with clear business implications is infinitely more valuable than a statistically superior 7-cluster solution that confuses everyone making actual decisions.
The perfect number of clusters is the one your organization can operationalize.
Name Your Clusters or Watch Them Die
Numbers don’t inspire action—stories do. After identifying clusters, you must translate cold statistics into compelling narratives.
When a luxury retailer discovered a critical customer segment, they didn’t call it “Cluster 3″—they named it “Aspirational Achievers,” complete with personas and journey maps that made the segment feel like real people.
Validation is non-negotiable. Use qualitative research to bring statistical clusters to life. Some of the most successful projects combine K-means cluster analysis in market research with focused interviews to validate and enrich understanding of each segment.
Refresh Regularly, But Not Obsessively
Markets evolve, and so should your understanding of them. Major clustering refreshes every 18-24 months work for most industries, with more frequent updates in rapidly changing sectors.
The magic of K-means cluster analysis in market research isn’t just finding patterns—it’s building organizational muscle memory around those patterns over time. That requires stability.
K-means Cluster Analysis in Market Research: Common Challenges and Solutions
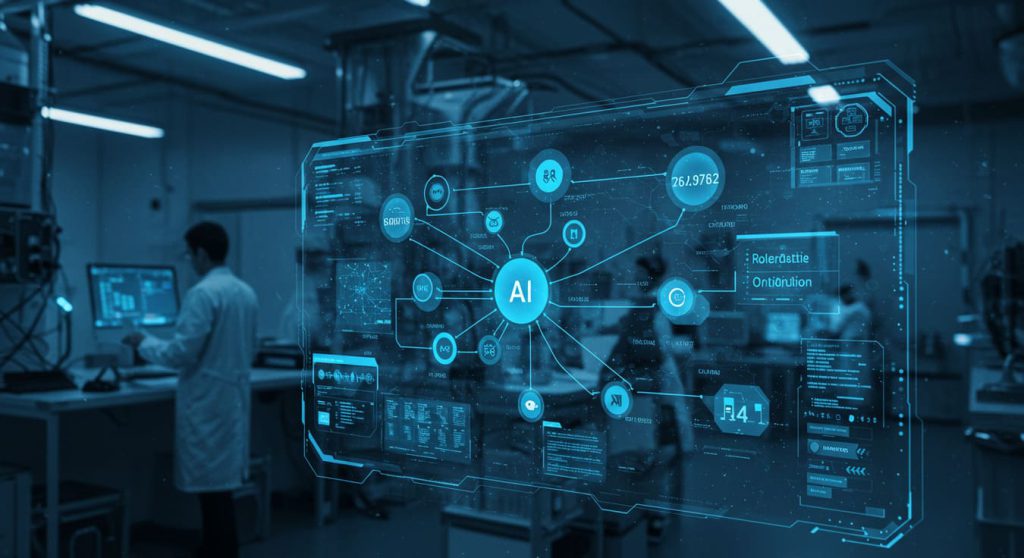
The most successful clustering projects don’t just run algorithms—they partner with organizations to ensure clustering drives tangible business results.
Let’s face it—implementing K-means cluster analysis in market research isn’t all rainbows and unicorns. Here are the obstacles most organizations face and how to overcome them:
The “Dirty Data” Dilemma
Garbage in, garbage out isn’t just a cliché—it’s the silent killer of clustering projects. Missing values, outliers, and inconsistent data formats will transform your beautiful analysis into an expensive hallucination.
Solution: Implement ruthless data preparation protocols before clustering begins. A healthcare client spent three painful weeks cleaning their customer dataset, which felt excessive until the resulting clusters revealed a patient segment worth $3.2 million in untapped annual revenue.
The “How Many Clusters?” Headache
Selecting the optimal number of clusters isn’t purely scientific—it requires business judgment alongside statistical validation.
Solution: Use a combination of technical approaches (elbow method, silhouette analysis) and business validation. For a retail banking client, presenting three clustering solutions (4, 6, and 8 segments) with explicit pros and cons of each allowed leadership to select the 6-segment solution that balanced nuance with actionability.
The “Interpretation Inertia” Issue
Even perfect clusters are worthless if your team stares at them like hieroglyphics.
Solution: Develop a “cluster translation” workshop where analysts, marketers, and executives collectively name and characterize each segment, creating shared language that drives aligned action. When teams create the narrative together, adoption skyrockets.
The “So What?” Syndrome
The most mathematically elegant clustering means absolutely nothing without business impact.
Solution: Before touching the data, define specific business decisions the clustering will inform. For a hospitality client, mapping exactly how cluster insights would influence pricing, promotion, and property development choices resulted in a 17% RevPAR increase within one year.
K-means cluster analysis in market research isn’t just a statistical technique—it’s a business transformation tool when applied correctly. The methodologies that bridge the gap between data science and business impact ensure that the patterns discovered drive tangible results.
Summary: K-means Cluster Analysis in Market Research
✅ K-means cluster analysis in market research reveals natural customer groupings that conventional segmentation approaches completely miss, potentially uncovering high-value segments hiding in plain sight within your data.
✅ Unlike demographic segmentation, K-means clustering identifies patterns based on actual behaviors, preferences, and needs, creating actionable intelligence rather than comfortable stereotypes.
✅ Successful implementation requires fanatically clean data, thoughtful variable selection, and a pragmatic balance between statistical rigor and business applicability.
✅ The optimal number of clusters balances mathematical validity with practical business application—usually between 3-8 segments for most market research applications.
✅ K-means cluster analysis has transformed results across industries, from retail to healthcare to financial services, with typical ROI improvements of 20-40% when properly implemented.
✅ Regular refreshes (typically every 18-24 months) keep clustering relevant while allowing sufficient time for organizational adoption and strategy implementation.
✅ The greatest challenge isn’t performing the analysis but translating statistical segments into compelling customer stories that drive aligned organizational action.
What Makes SIS International a Top K-Means Cluster Analysis in Market Research Provider?
The approach to K-means cluster analysis that delivers exceptional results combines statistical rigor with business pragmatism. Here’s what separates transformative clustering projects from expensive disappointments:
✔ GLOBAL REACH: Researchers in 120+ countries capture cultural nuances that affect clustering validity. A consumer electronics clustering project identified crucial regional variations that led to a 31% improvement in international marketing performance by recognizing that behavioral patterns varied significantly across cultural contexts.
✔ 40+ YEARS OF EXPERIENCE: Since 1984, market research has evolved through multiple paradigm shifts. K-means cluster analysis techniques have been refined through hundreds of implementations across industries. This depth of experience means problems can be anticipated before they derail projects.
✔ GLOBAL DATABASES FOR RECRUITMENT: Access to over 53 million research participants worldwide ensures clustering based on robust, representative samples. A recent pharmaceutical segmentation accessed specialized participants across 8 countries in just 10 days, something competitors quoted 6+ weeks to accomplish.
✔ IN-COUNTRY STAFF WITH OVER 33 LANGUAGES: Clustering requires nuanced understanding of customer motivation that often gets lost in translation.
✔ GLOBAL DATA ANALYTICS: The most effective projects combine K-means with other advanced techniques like random forests and neural networks when appropriate, creating hybrid approaches that extract maximum insight from data.
✔ AFFORDABLE RESEARCH: Sophisticated doesn’t mean unaffordable. Efficient global structures allow enterprise-grade clustering at price points accessible to mid-market companies. Even startups with limited budgets can achieve segmentation insights previously available only to Fortune 500 corporations.
✔ CUSTOMIZED APPROACH: Cookie-cutter methodologies fail. When standard K-means clustering wasn’t capturing the complexity of a fashion retailer’s customer base, a custom two-stage clustering approach revealed crucial subsegments driving 27% of their profit from just 8% of customers.
Frequently Asked Questions About K-Means Cluster Analysis in Market Research
What exactly makes K-means different from traditional market segmentation?
Traditional segmentation starts with predetermined categories (like demographics) and forces customers into these boxes. K-means cluster analysis in market research does the opposite—it lets natural patterns emerge from data without preconceptions. This often reveals surprising customer groupings based on actual behaviors and preferences rather than assumptions. This approach consistently uncovers high-value segments that traditional methods miss entirely.
How much data do we need for effective K-means clustering?
While more data generally improves clustering quality, it’s not just about quantity. Successful projects have worked with as few as 300 respondents and others with millions. The critical factor is having the right variables that capture meaningful differences between customer groups. Quality absolutely decimates quantity every time.
The right 10 variables will reveal more insights than the wrong 100 variables.
Can K-means clustering help with new product development?
Absolutely—it’s one of the most powerful applications! K-means clustering helps you avoid the “average product for average customers” trap by identifying distinct customer segments with different needs.
How often should we update our customer clusters?
It depends on your industry’s rate of change. In fast-moving sectors like technology or fashion, refreshing clusters every 12-18 months is prudent. In more stable industries, every 2-3 years may suffice. The key is balancing freshness with operational consistency—your organization needs time to implement strategies based on the segments before you change them.
Can K-means clustering work with qualitative data?
While K-means naturally works with numerical data, techniques exist to incorporate qualitative insights. Converting qualitative data into quantifiable variables or using hybrid approaches that combine K-means with other methodologies often yields powerful results.
How do we translate statistical clusters into actionable marketing strategies?
This is where art meets science. Once statistically valid clusters are identified, deep-dive analysis creates rich profiles of each segment, including behaviors, attitudes, pain points, and purchase drivers. Workshops with cross-functional teams develop tailored strategies for each segment.
Numbers don’t drive action. Stories do.
Is K-means cluster analysis worth the investment for smaller businesses?
Companies of all sizes benefit from K-means clustering, but the approach often differs. For smaller businesses, focused clustering around specific business questions rather than comprehensive customer segmentation delivers exceptional ROI. Sometimes, the smallest companies have the most to gain because they can adapt faster to the insights revealed.
The right question is worth a thousand answers. K-means cluster analysis in market research helps you discover questions about your market you didn’t even know to ask—and that’s where breakthrough insights begin.
Nuestra ubicación de instalaciones en Nueva York
11 E 22nd Street, Piso 2, Nueva York, NY 10010 T: +1(212) 505-6805
Acerca de SIS Internacional
SIS Internacional ofrece investigación cuantitativa, cualitativa y estratégica. Proporcionamos datos, herramientas, estrategias, informes y conocimientos para la toma de decisiones. También realizamos entrevistas, encuestas, grupos focales y otros métodos y enfoques de investigación de mercado. Póngase en contacto con nosotros para su próximo proyecto de Investigación de Mercado.