Advanced Customer Segmentation
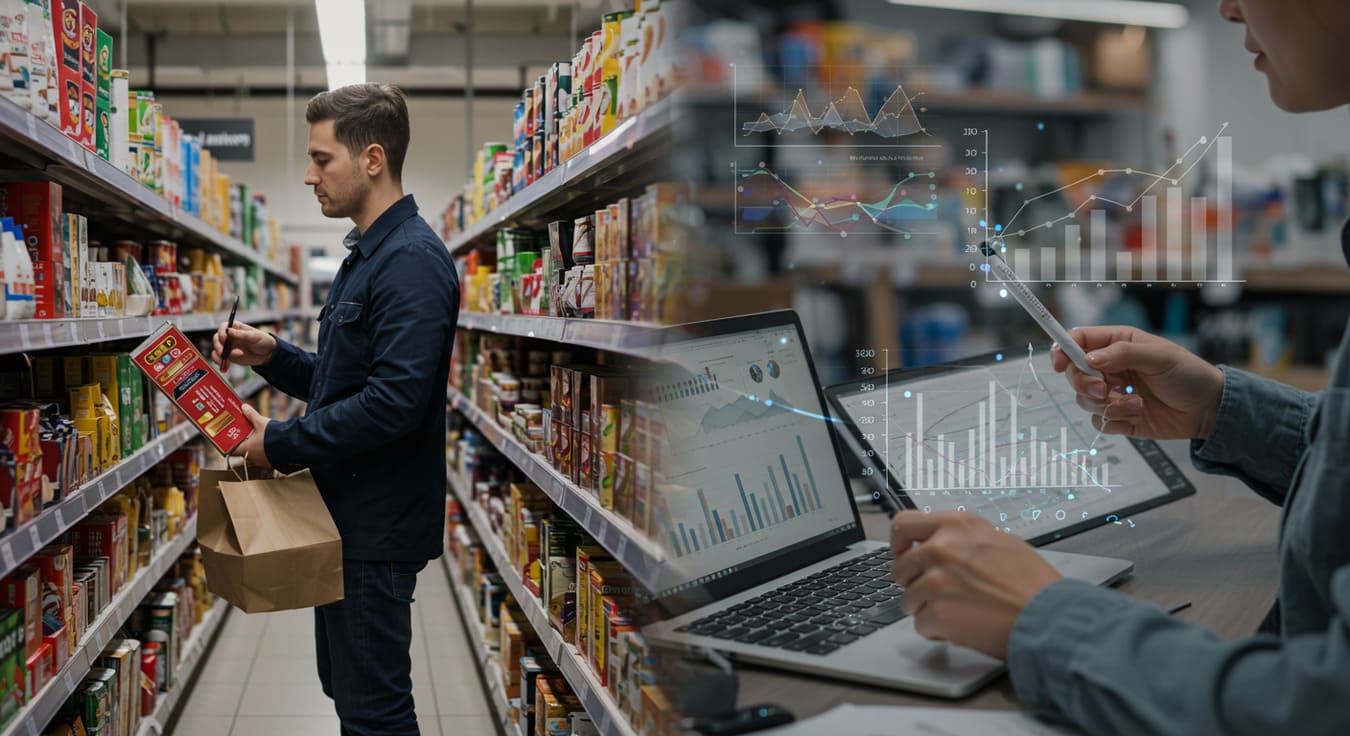
Traditional customer segmentation is dead, and most marketers haven’t even noticed the funeral.
At least 80% of the segmentations being used by major brands today are embarrassingly outdated – digital paperweights that create the illusion of customer understanding while actually driving companies further from market reality.
Let me show you why traditional segmentation is increasingly a recipe for market irrelevance – and how companies dominating their categories have embraced something radically different.
Table of Contents
✅ Listen to this PODCAST EPISODE here:
What is Advanced Customer Segmentation?
Advanced customer segmentation isn’t just a better version of traditional segmentation—it’s a completely different animal.
Traditional segments typically rely on simplistic demographic variables (age, income, zip code) with perhaps a sprinkle of purchase history. It’s marketers playing connect-the-dots with only five dots and somehow expecting to see the Mona Lisa.
Advanced customer segmentation, by contrast, integrates multiple dimensions of behavioral, psychographic, predictive, and engagement data to identify patterns that actually drive business outcomes, not just look pretty in board decks.
This distinction isn’t academic—it’s existential. Particularly, advanced customer segmentation differs from traditional approaches in several critical ways:
- It’s multi-dimensional rather than flat
- It’s behavior-focused rather than assumption-driven
- It’s predictive rather than just descriptive
- It’s dynamic rather than frozen in time
- It’s opportunity-oriented rather than merely checking a marketing box
Key Methodologies in Advanced Customer Segmentation
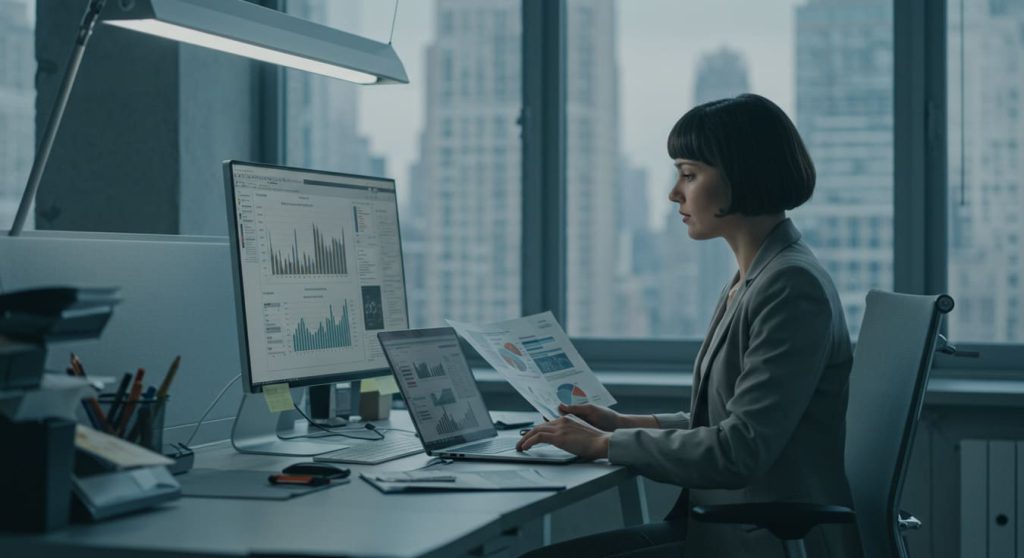
This isn’t just better targeting. It’s a fundamentally different understanding of your market.
The most powerful advanced customer segmentation implementations don’t rely on a single methodology—they integrate multiple approaches to create multi-dimensional understanding that competitors can’t easily replicate.
Let’s skip the consultant-speak and examine what actually delivers results:
Behavioral Segmentation Approaches
Behavioral segmentation focuses on what customers actually do rather than who marketing assumes they are. This includes:
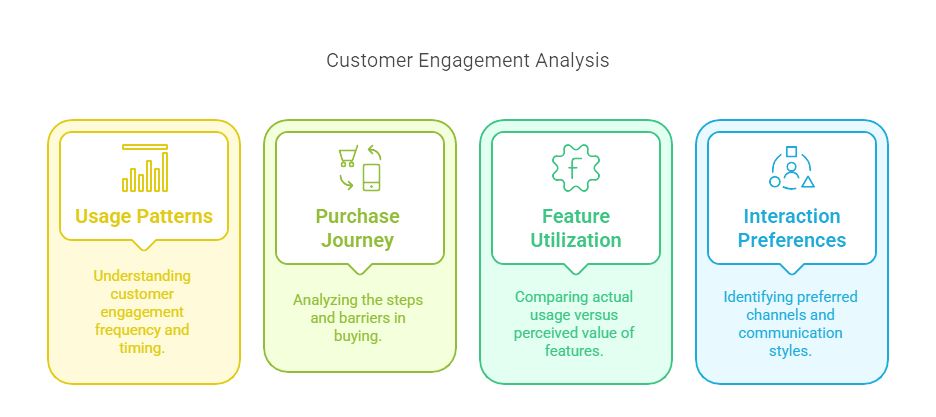
- Usage patterns: How, when, and how often customers engage with products/services
- Purchase journey analysis: The steps, triggers, and barriers in the buying process
- Feature/benefit utilization: Which aspects of offerings customers actually use versus what you think they value
- Interaction preferences: How customers prefer to engage (channels, frequency, tone)
A software company was genuinely shocked when behavioral segmentation revealed their “power users” fell into three fundamentally different groups with completely different feature utilization patterns. What they thought was one segment was actually three distinct groups using their product for entirely different purposes.
This wasn’t just an interesting insight—it forced them to redesign their entire product roadmap and drastically reduced churn from 18% to 7% annually.
Psychographic Analysis Techniques
Psychographic segmentation digs beneath surface behaviors to examine the psychological drivers behind customer choices:
- Values and beliefs: Core principles that influence decisions
- Lifestyle patterns: How customers spend time and prioritize activities
- Aspirations and goals: What customers are trying to achieve
- Social identification: How customers view themselves in relation to others
For example, a consumer packaged goods client was hemorrhaging market share until psychographic analysis identified a segment they named “Practical Environmentalists”—consumers deeply concerned about sustainability but unwilling to pay premium prices that existing “green” brands demanded.
This insight led to a new product line that delivered eco-friendly benefits at mainstream price points, capturing $43 million in first-year sales from competitors who were still segmenting solely by income and education levels.
Predictive Value-Based Segmentation
Value-based segmentation focuses on current and potential future value—because not all customers deserve equal investment:
- Customer lifetime value (CLV) modeling: Projecting long-term value based on behavioral indicators
- Share-of-wallet analysis: Identifying spending potential by category versus competitors
- Propensity modeling: Predicting likelihood of specific high-value behaviors
- Risk/churn prediction: Identifying vulnerability patterns before customers themselves decide to leave
A hospitality company implemented predictive value-based segmentation and discovered something their executives found hard to believe: their marketing was over-invested in a high-volume but low-margin segment while under-investing in a smaller segment with 3.7x higher lifetime value.
The head of marketing initially rejected this finding until we showed him the raw transaction data. Reallocating just 20% of their marketing budget increased overall profitability by 28% in the first three quarters.
Hybrid Multi-Factor Models
The most sophisticated implementations integrate multiple segmentation dimensions to create proprietary views of the market that competitors can’t easily copy:
- Behavior-value matrices: Combining behavioral patterns with value metrics to prioritize initiatives
- Needs-based hybrid models: Integrating functional and emotional needs to drive product innovation
- Life-stage value frameworks: Mapping behavior changes through customer evolution to predict next actions
- Dynamic engagement models: Adapting segments based on interaction patterns in real-time
For instance, a financial services provider created a hybrid segmentation that integrated behavioral, value, and life-stage dimensions. This allowed them to identify specific trigger points where customers became receptive to new product categories—increasing cross-sell conversion by 64% compared to their traditional age-and-assets approach.
The fatal mistake many organizations make is treating advanced customer segmentation as a one-dimensional exercise. The real power comes from integration—behavioral patterns that reveal psychological drivers that predict future value that informs prioritization decisions.
Implementation Roadmap for Advanced Segmentation
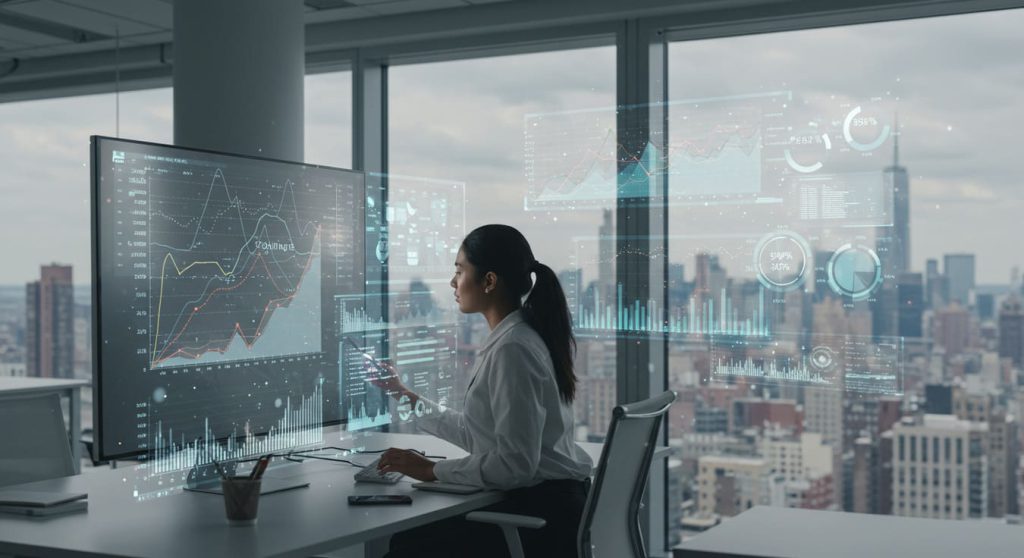
Moving from concept to implementation is where most advanced customer segmentation initiatives succeed or fail… And these clear patterns separate transformative successes from expensive disappointments.
Data Infrastructure Requirements
Advanced segmentation demands thoughtful data architecture from day one:
- Customer data platform (CDP): Unified customer data repository with identity resolution capabilities
- Real-time data processing capabilities: Enabling dynamic segmentation updates rather than quarterly refreshes
- Dimensional data access: Supporting exploration across multiple variable combinations without IT bottlenecks
- Sufficient historical depth: Typically 18-36 months of behavioral data to identify stable patterns
Analytical Capability Development
Advanced customer segmentation requires specific analytical competencies beyond traditional market research skills:
- Unsupervised learning techniques: Cluster analysis, factor analysis, dimensional reduction
- Supervised modeling approaches: Propensity modeling, value prediction, churn probability
- Visualization capabilities: Translating complex patterns into understandable insights for non-technical users
- Testing frameworks: Validating segment distinctiveness and stability over time
Cross-Functional Integration Steps
Segmentation that stays within the analytics department creates exactly zero value:
- Insight translation workshops: Converting analytical outputs to business understanding for different functions
- Segment immersion programs: Helping teams understand segments as people, not abstract categories
- Operational integration planning: Mapping precisely how segments will influence specific decisions
- Capability gap assessment: Identifying what teams need to actually act on segment insights
Testing and Validation Processes
Rigorous validation isn’t a nice-to-have option—it’s non-negotiable:
- Statistical validity testing: Ensuring segments are meaningfully distinct versus random groupings
- Temporal stability analysis: Confirming segments persist over time rather than being temporary artifacts
- Predictive power verification: Testing whether segments actually predict important business outcomes
- Business impact modeling: Quantifying potential value of segment-based strategies before full implementation
Organizational Change Management
The human element often determines success or failure more than technical factors:
- Executive sponsorship cultivation: Securing senior-level commitment to segment-based approaches
- Success metrics alignment: Agreeing how segmentation impact will be measured before implementation
- Capability building programs: Training teams to use segment insights effectively in daily decisions
- Incentive alignment: Ensuring rewards support segment-based strategies rather than contradicting them
Common Challenges and Solutions in Advanced Segmentation
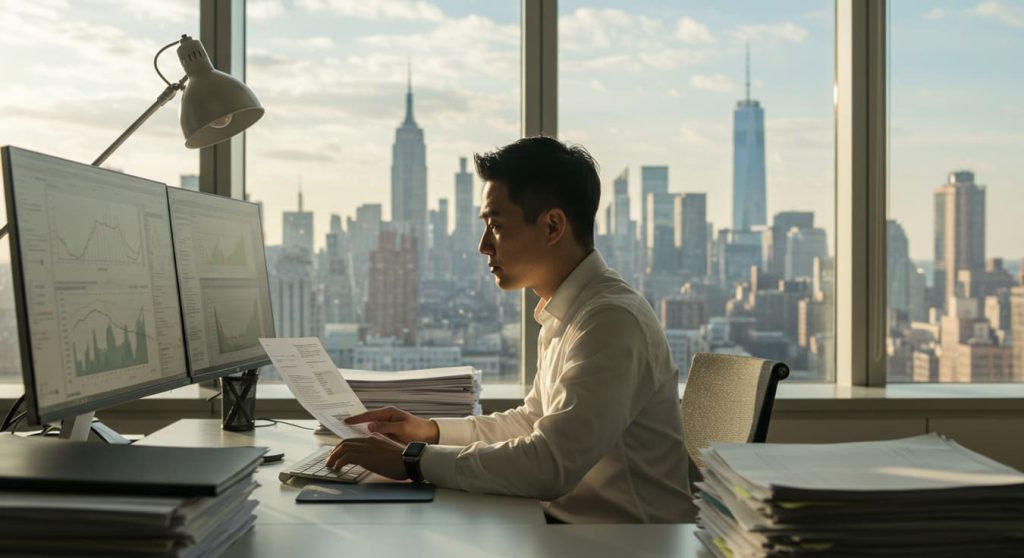
Let’s address the toughest obstacles in implementing advanced customer segmentation and how to overcome them:
Data Quality and Integration Issues
The most sophisticated segmentation will fail spectacularly with poor-quality data:
✔ Challenge: Inconsistent data collection, siloed data sources, incomplete customer views
✔ Impact: Segments based on partial data create distorted strategies
✔ Solution: A consumer products company created a “data quality scorecard” that evaluated completeness, accuracy, and integration quality for each data source. They prioritized fixing the highest-impact quality issues first, focusing on variables most critical to their specific segmentation objectives. This pragmatic approach improved their key data quality metrics from 62% to 91% within six months.
The VP of Analytics summed it up perfectly: “We stopped trying to boil the ocean and focused on making the specific improvements that would actually impact our segmentation.”
✔ Challenge: Insufficient historical data for pattern identification
✔ Impact: Segments reflect recent anomalies rather than stable patterns
✔ Solution: An e-commerce company lacking sufficient historical data implemented progressive profiling—collecting additional customer information through staged interactions rather than all at once. This approach increased their data completeness by 43% within 90 days while maintaining conversion rates.
Their chief digital officer noted: “We realized we didn’t need perfect data on day one. We needed a process to continuously improve data quality while still making better decisions with what we had.”
Analytical Complexity Barriers
Advanced customer segmentation can become prohibitively complex without proper guardrails:
✔ Challenge: Over-engineered segmentations with too many variables and segments
✔ Impact: Models that are statistically impressive but operationally useless
✔ Solution: A financial services client simplified their initial 67-variable segmentation model to 14 high-impact variables, actually improving predictive accuracy by 11% while dramatically increasing usability. Their mantra became “complex understanding, simple application.”
Their head of customer experience observed: “We initially thought more variables meant better segmentation. We were dead wrong. Fewer, better-chosen variables created segments our teams could actually understand and use.”
✔ Challenge: Difficulty explaining advanced methodologies to business users
✔ Impact: Limited adoption due to mistrust of “black box” approaches
✔ Solution: A telecommunications provider created a “segmentation translation layer” that converted complex statistical outputs into business-friendly visualizations and narratives. This approach increased segment understanding among business users from 23% to 89% based on their internal assessment.
Their SVP of Marketing admitted: “I initially rejected the segmentation because I couldn’t explain it to my team. The visualization tools changed everything—suddenly we could see the patterns that the algorithms were finding.”
Operationalizing Insights Across Organizations
Even perfect segmentations fail without operational implementation:
✔ Challenge: Difficulty translating segment insights into channel-specific actions
✔ Impact: Segments that influence planning but not execution
✔ Solution: A retailer developed segment playbooks for each customer-facing function (marketing, merchandising, store operations, customer service), translating the same segment insights into channel-relevant actions. This approach increased segment-aligned actions by 310% within six months.
Their chief customer officer noted: “We realized marketing, stores, and digital teams needed different versions of the same segment truth. Once we translated the insights into function-specific guidelines, adoption skyrocketed.”
✔ Challenge: Legacy systems unable to act on dynamic segmentation
✔ Impact: Analytical sophistication constrained by operational limitations
✔ Solution: A travel company unable to fully integrate advanced segmentation into legacy systems implemented a phased approach, starting with marketing campaigns (easiest to adapt) while building business case for system upgrades based on initial results. Their 43% improvement in marketing performance funded subsequent technology investments.
Their CIO later shared: “We stopped making perfect the enemy of better. By showing concrete results with what we could implement immediately, we built the case for the bigger systems changes we ultimately needed.”
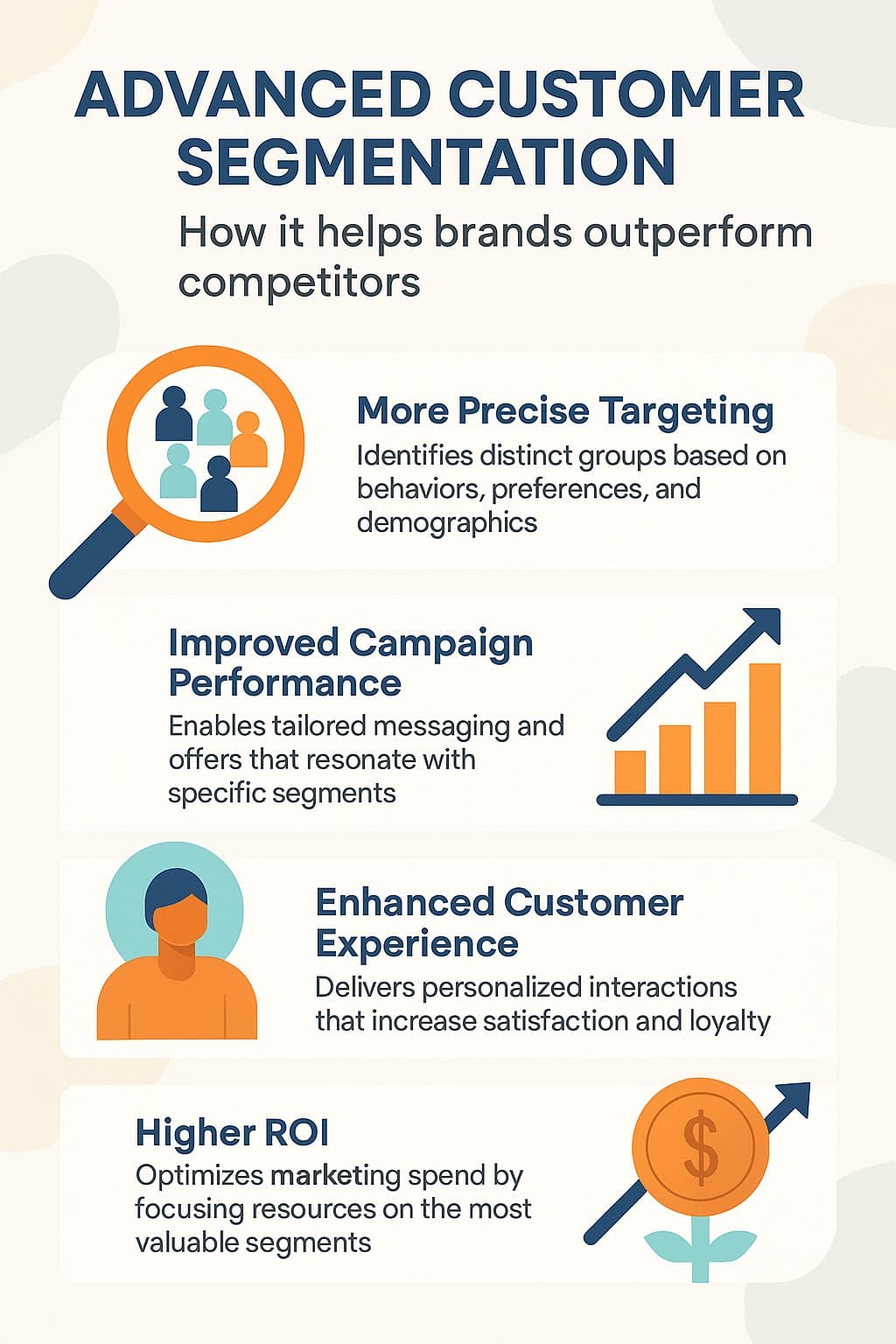
Key Insights Summary
✅ Advanced customer segmentation integrates behavioral, psychographic, and value dimensions to reveal meaningful patterns traditional demographic approaches miss entirely.
✅ The most effective segmentations combine multiple methodologies (behavioral, psychographic, predictive, and value-based) to create multi-dimensional customer understanding that’s difficult for competitors to replicate.
✅ Data integration across sources (digital footprints, transactions, engagement metrics, and sentiment) provides the foundation for discovering non-obvious customer patterns that drive disproportionate business impact.
✅ Implementation success depends as much on organizational readiness and operational integration as on analytical sophistication—the best model never implemented creates zero value.
✅ Common implementation barriers include data quality issues, analytical complexity, operational limitations, and measurement challenges—all of which have proven solutions that successful companies have deployed.
✅ The ROI potential from advanced customer segmentation typically ranges from 30-70% improvement in marketing performance and 15-35% increase in customer lifetime value when properly implemented across functions.
✅ The most successful organizations treat segmentation as a strategic capability rather than a tactical marketing tool, integrating segment insights across all customer-facing functions.
What Makes SIS International a Top Advanced Segmentation Provider?
À SIS, we deliver exceptional results today, combining analytical sophistication with pragmatic business integration.
✔ GLOBAL REACH: With researchers across 120+ countries, cultural nuances that affect segmentation validity can be captured and incorporated.
✔ 40+ YEARS OF EXPERIENCE: Since 1984, segmentation methodologies have evolved through multiple paradigms. Advanced customer segmentation approaches have been refined through hundreds of implementations across industries, with each iteration improving both analytical power and business integration.
✔ GLOBAL DATA BASES FOR RECRUITMENT: Access to over 53 million research participants worldwide ensures segmentation models based on robust, representative samples.
✔ IN-COUNTRY STAFF WITH OVER 33 LANGUAGES: Effective segmentation requires nuanced understanding of cultural context that often gets lost in translation.
✔ GLOBAL DATA ANALYTICS: The most effective projects integrate advanced customer segmentation with complementary analytical approaches, creating methodologies that maximize insight discovery.
✔ AFFORDABLE RESEARCH: Sophisticated segmentation doesn’t require Fortune 500 budgets. Efficient global structures allow enterprise-grade analytics at midmarket price points. Even growth-stage companies can access segmentation insights previously available only to industry giants.
Frequently Asked Questions About Advanced Customer Segmentation
How does advanced customer segmentation differ from traditional approaches?
Traditional segmentation typically relies primarily on demographics and broad purchase history, creating simplistic groupings like “millennial professionals” or “suburban households with children.” Advanced customer segmentation integrates behavioral patterns, psychographic drivers, predictive indicators, and value metrics to identify meaningful distinctions traditional approaches miss entirely.
What data is required for effective advanced segmentation?
While more data generally improves segmentation quality, the critical factor is having the right variables that capture meaningful differences between customer groups. The essential data components typically include:
- Behavioral data (purchases, browsing, engagement patterns)
- Value metrics (current and potential value indicators)
- Psychographic information (preferences, priorities, attitudes)
- Engagement history (response patterns, channel interactions)
How many segments should we create?
The optimal number of segments balances actionability against market coverage. While statistical methods like scree plots or silhouette analysis provide technical guidance, business factors are equally important:
- Operational capacity to deliver differentiated approaches
- Meaningful performance differences between segments
- Sufficient segment size to justify specialized treatment
- Strategic priorities and resource constraints
How often should we update our customer segmentation?
Market dynamics and internal capabilities should determine your refresh cadence:
- High-volatility markets typically require annual refreshes
- Moderate-volatility industries may update every 18-24 months
- Stable industries might refresh every 2-3 years
- Major market disruptions should trigger immediate reassessment
How do we measure the ROI of advanced customer segmentation?
Effective measurement requires both direct and indirect metrics:
- Direct performance metrics: Campaign response, conversion rates, average order value
- Value-based indicators: Customer lifetime value, retention rates, share of wallet
- Operational efficiency measures: Acquisition costs, service costs, marketing efficiency
- Strategic value creation: New product adoption, category expansion, advocacy metrics
Notre emplacement à New York
11 E 22nd Street, étage 2, New York, NY 10010 Tél. : +1(212) 505-6805
À propos de SIS International
SIS International propose des recherches quantitatives, qualitatives et stratégiques. Nous fournissons des données, des outils, des stratégies, des rapports et des informations pour la prise de décision. Nous menons également des entretiens, des enquêtes, des groupes de discussion et d’autres méthodes et approches d’études de marché. Contactez nous pour votre prochain projet d'étude de marché.