How SIS Uses AI Methods for Product Testing
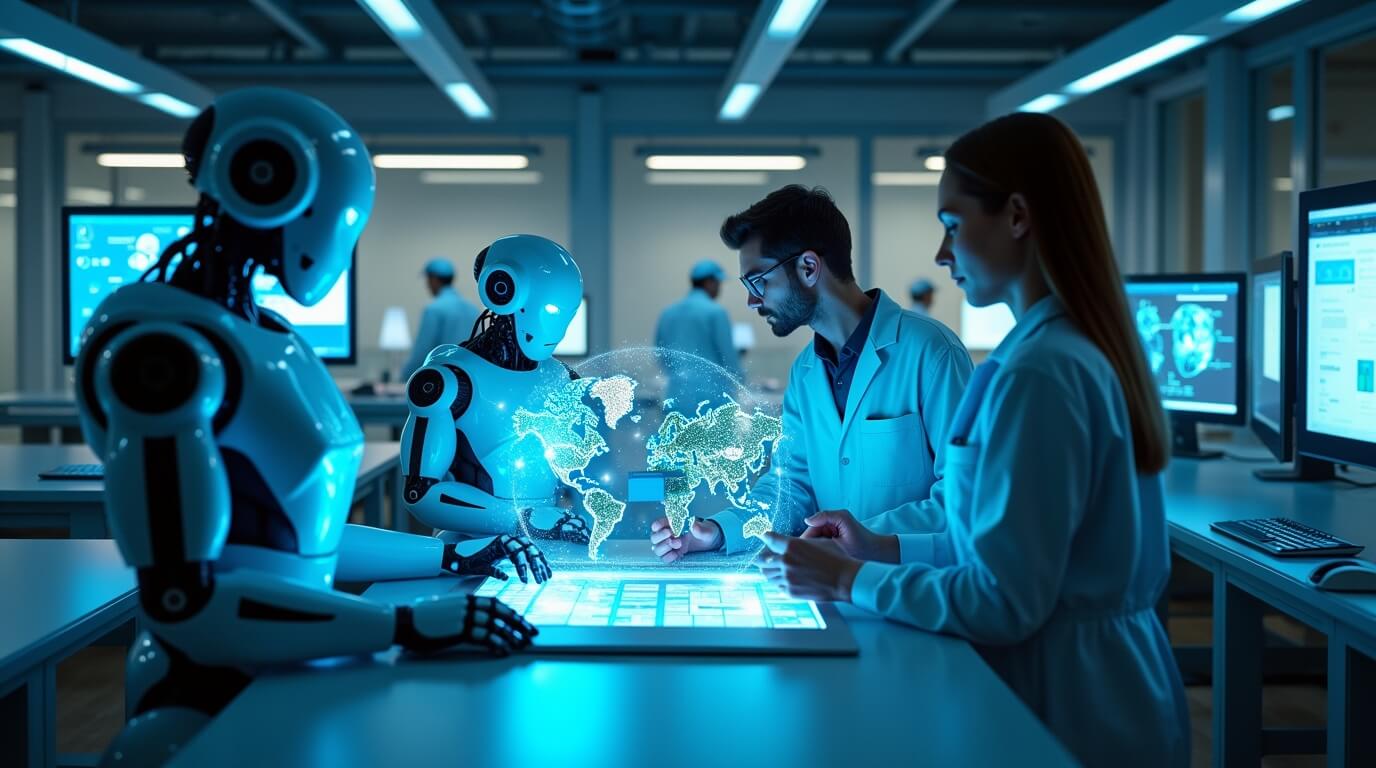
The organizations embracing sophisticated AI methods for product testing are gaining compound advantages that extend far beyond incrementally better testing results.
My smartwatch can detect a subtle heart rhythm abnormality months before any symptoms appear. How? Through sophisticated AI analysis that spotted patterns invisible to human observation. At SIS Internacional, we’ve applied similar AI methods for product testing that revolutionize how brands validate their offerings before market launch.
You interact daily with products shaped by AI testing methods without ever realizing it. We’ve pioneered AI methods for product testing that don’t just identify existing problems—they predict potential issues before they occur.
The Evolution of AI in Product Testing
When we first began exploring AI methods for product testing at SIS, we faced considerable skepticism. Many clients couldn’t understand how algorithms could outperform human inspectors with decades of experience. That skepticism vanished when our AI methods for product testing identified a subtle material interaction issue that had eluded detection through six months of conventional testing.
The evolution of AI methods for product testing has accelerated dramatically in recent years. Early applications focused primarily on automating visual inspection tasks, using computer vision to identify defects more consistently than human inspectors. Our specialists have documented how AI methods for product testing are particularly valuable for complex products with numerous potential failure modes. One electronics manufacturer told me, “Traditional testing required us to anticipate every possible problem scenario. AI methods identify issues we never would have thought to test for.” This shift from hypothesis-driven to discovery-based testing represents a fundamental advantage of AI methods for product testing.
Furthermore, the ROI from implementing sophisticated AI methods for product testing has consistently exceeded our clients’ expectations. Beyond catching defects, these approaches shorten development cycles, reduce testing costs, and enable more innovative designs by providing faster feedback loops.
Computer Vision: Beyond the Human Eye
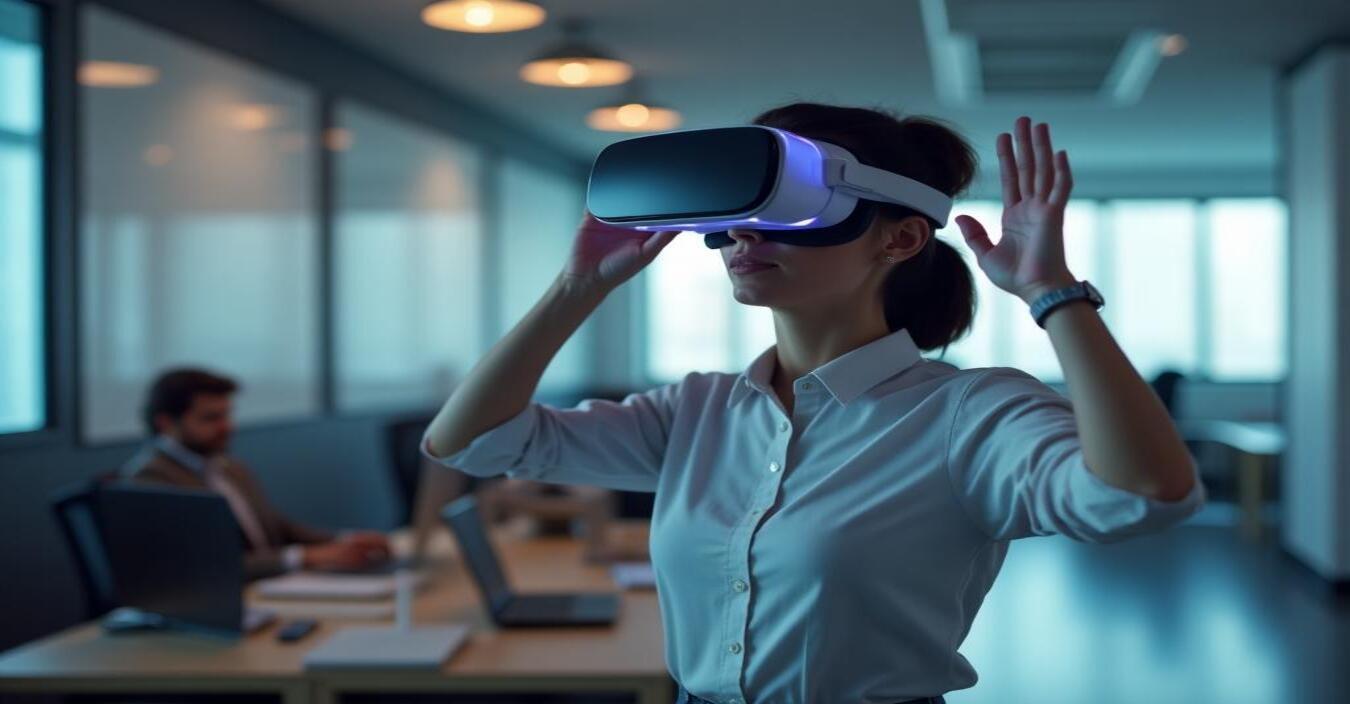
Advanced computer vision-based AI methods for product testing can detect defects as small as 0.01mm—far below the threshold of human visual perception. We’ve implemented these systems for clients in industries from pharmaceuticals to electronics, where microscopic flaws can have major consequences.
Additionally, our analysis shows that conventional visual inspection accuracy typically drops 28% after four hours, while AI methods for product testing maintain consistent performance indefinitely. This reliability factor is why many of our clients have made computer vision AI the cornerstone of their quality programs.
The speed capabilities of modern AI methods for product testing have enabled 100% inspection in production environments where sampling was previously the only option. Systems we’ve implemented can analyze hundreds of products per minute with accuracy exceeding manual inspection. This transition from statistical quality control to comprehensive verification represents a fundamental shift enabled by AI methods for product testing.
Predictive Analytics: Forecasting Performance and Failures
Beyond inspection, AI methods for product testing have transformed our ability to predict how products will perform in the real world before they ever leave the laboratory.
Traditional testing examines one factor at a time, missing complex interactions that AI methods for product testing readily identify. One automotive components client discovered through our AI-powered analysis that a failure mode they had attributed to temperature was actually caused by a subtle interaction between temperature, humidity, and vibration frequency—an insight that would have been virtually impossible to discover through conventional testing.
These AI methods for product testing are particularly valuable for reliability engineering. We recently helped a consumer electronics manufacturer implement AI methods for product testing that reduced their reliance on lengthy lifecycle testing, cutting six weeks from their development timeline while actually improving reliability prediction accuracy.
Additionally, the anomaly detection capabilities of AI methods for product testing have proven especially valuable for identifying outliers that might indicate potential issues. Traditional statistical methods typically focus on averages and standard deviations, potentially missing important signals. Advanced AI methods for product testing can identify subtle pattern deviations that merit further investigation.
Natural Language Processing: Understanding Customer Feedback
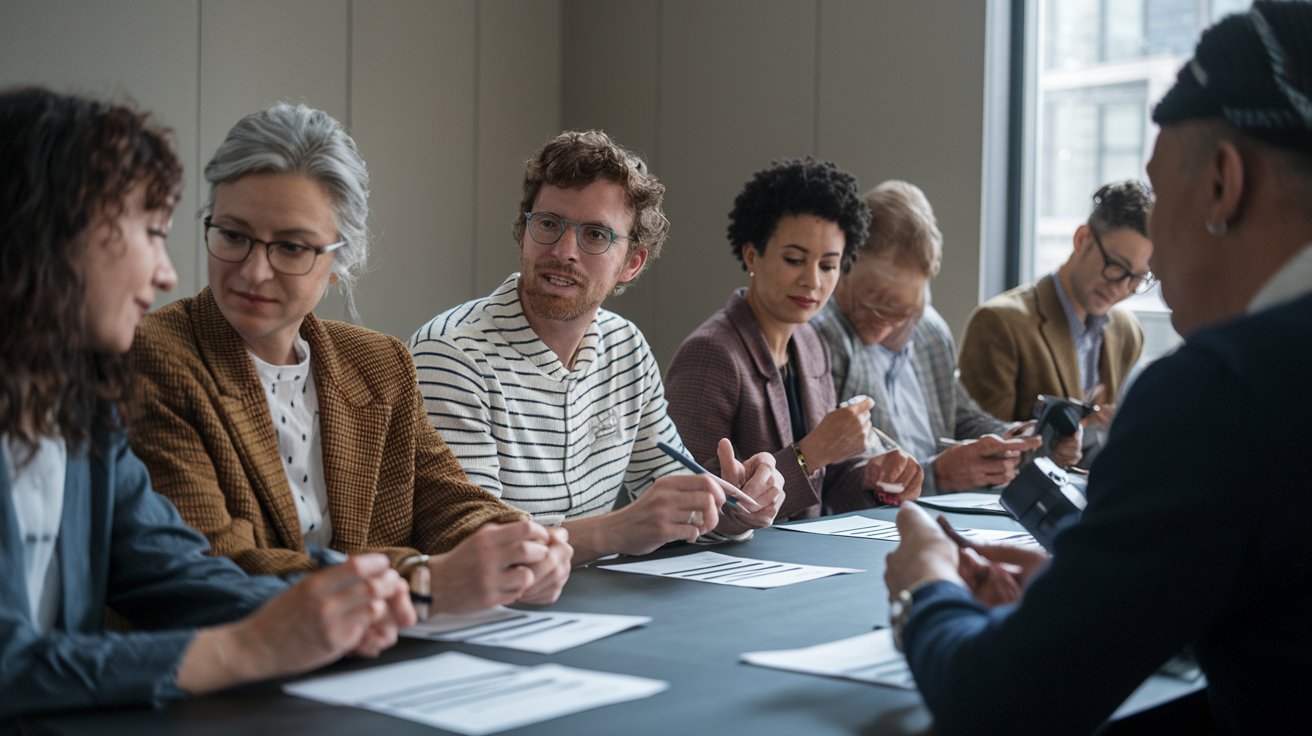
The voice of the customer has always been central to effective product testing. AI methods for product testing have transformed how we analyze and apply this critical feedback.
Natural language processing (NLP) AI methods for product testing can analyze thousands of customer reviews, support tickets, and social media mentions to identify emerging issues far earlier than traditional monitoring approaches. We’ve implemented systems that scan over 20 million social media posts monthly, using sophisticated AI methods for product testing to distinguish between random complaints and emerging patterns that indicate potential product issues.
The sentiment analysis capabilities of these AI methods for product testing go far beyond simple positive/negative classification. Advanced algorithms can identify specific emotional responses to product features, detecting nuances that would be missed in traditional surveys. One consumer goods executive was stunned when our AI methods for product testing revealed that a feature they considered minor was generating the strongest emotional response from customers—an insight that fundamentally changed their marketing emphasis.
Topic clustering algorithms represent another powerful application of AI methods for product testing. These approaches can organize unstructured feedback into coherent themes without predefined categories, revealing concerns that structured research might miss entirely.
Machine Learning in User Experience Testing
Understanding how users actually interact with products has been revolutionized by AI methods for product testing that can analyze behavioral patterns at scale.
The pattern recognition capabilities of machine learning AI methods for product testing can identify usage obstacles that users might not articulate. These systems detect where users hesitate, make errors, or abandon processes—insights that go beyond what users explicitly report.
Clustering algorithms represent another powerful application of AI methods for product testing for user experience analysis. These approaches identify different user behavior patterns, revealing how various user segments interact with products in distinct ways. We recently implemented AI methods for product testing for a consumer electronics client that identified five distinct usage patterns among their customers, allowing them to optimize the interface for multiple user types rather than designing for an “average user” that didn’t actually exist.
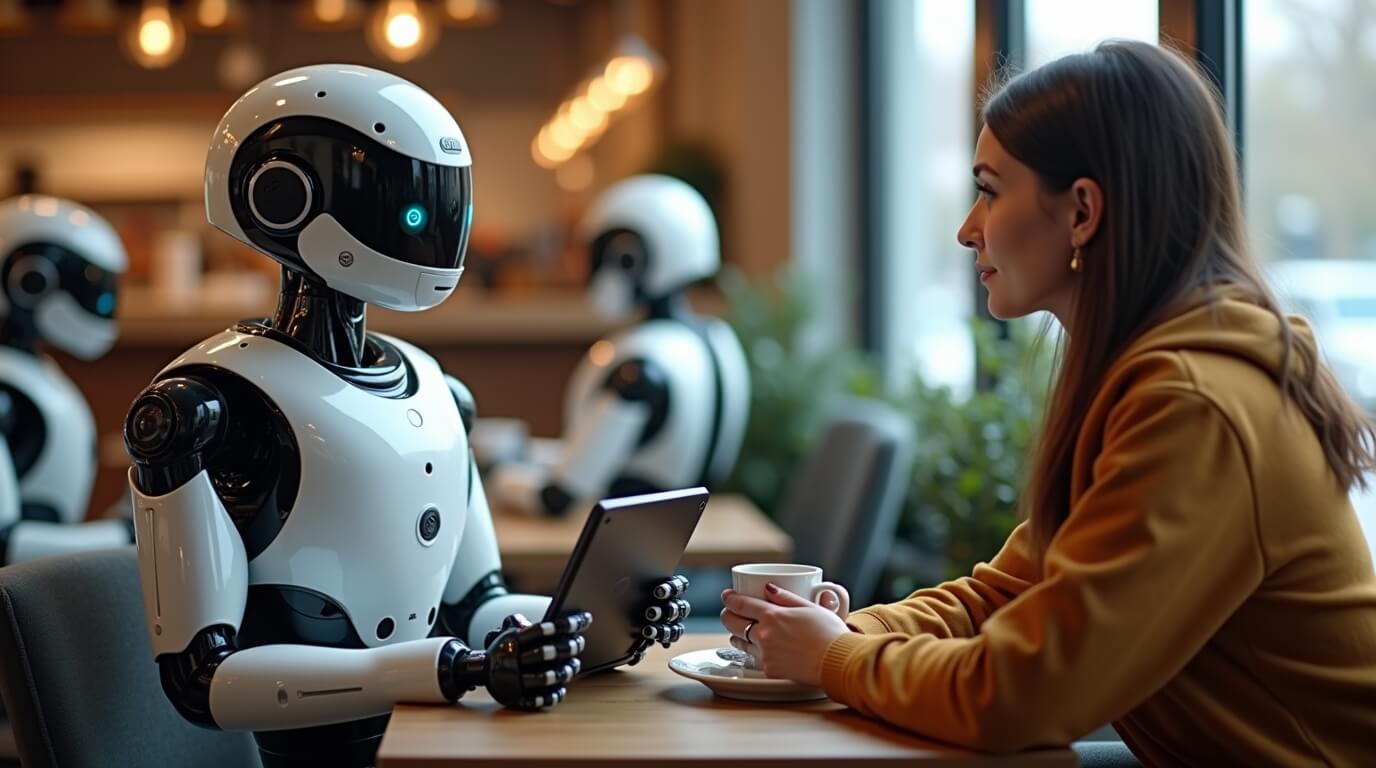
Anomaly detection in user testing applies AI methods for product testing to identify unusual interaction patterns that might indicate usability problems. Unlike traditional testing that typically focuses on average performance, these approaches specifically look for outliers and edge cases. One software client discovered through AI-powered analysis that 8% of users were following an unexpected, significantly less efficient navigation path—a pattern that wouldn’t have been apparent in conventional testing focused on common behaviors.
The Future of AI in Product Testing at SIS
As I look toward the horizon, several emerging developments in AI methods for product testing promise to further transform how SIS helps clients validate their products.
Edge AI represents one of the most promising frontiers for product testing. These approaches enable real-time analysis and decision-making without cloud connectivity by embedding AI capabilities directly into testing equipment. We’re pioneering AI product testing methods that allow sophisticated inspection and testing at remote manufacturing locations with limited infrastructure.
Quantum computing applications for AI methods for product testing are still emerging but show tremendous potential. These approaches promise to solve complex optimization problems that remain challenging for conventional computing. We’re collaborating with research partners to develop quantum-enhanced AI methods for product testing that could revolutionize simulation capabilities, potentially enabling virtual testing of complex systems with perfect fidelity to physical reality.
Generative AI represents another frontier in product testing. Beyond analyzing existing designs, these systems can propose modifications to address identified issues or optimize for specific performance parameters. We’re developing AI methods for product testing that don’t just identify problems but suggest specific design changes to resolve them.
At SIS International Research, we’re committed to pushing the boundaries of what’s possible with AI methods for product testing, helping our clients create products that don’t just meet expectations—they set new standards for quality, reliability, and user satisfaction. In a marketplace where excellence is the minimum requirement for survival, AI-powered testing may be your most powerful tool for sustainable competitive advantage.
Key Insights: AI Methods for Product Testing
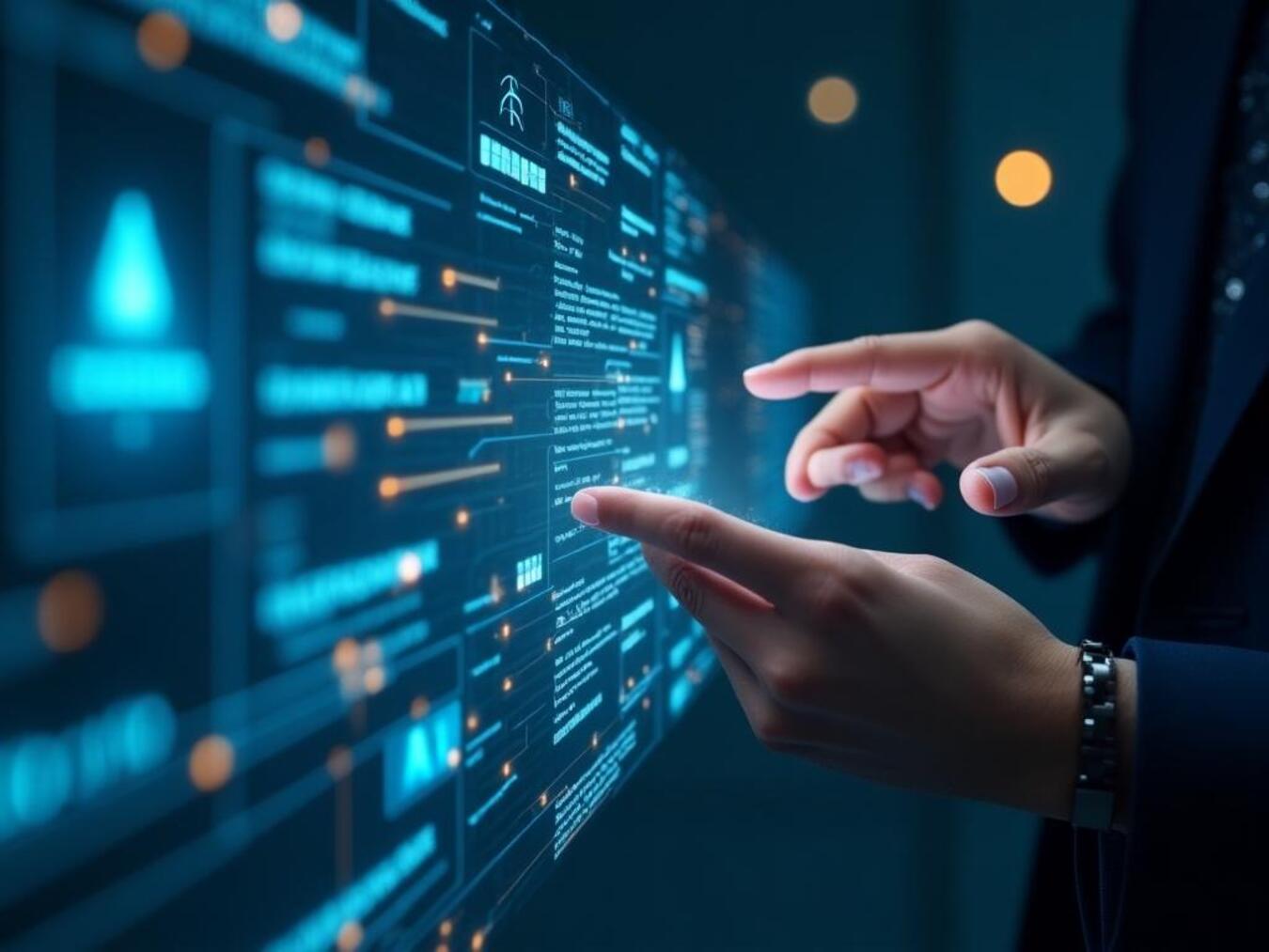
- Transformational capabilities – AI methods have evolved from simple automation to predictive, adaptive systems that can find patterns across massive datasets that are impossible for traditional analytics to process
- Computer vision advantages:
- Detection of defects as small as 0.01mm—far below human visual perception
- Consistent performance without fatigue (compared to 28% accuracy drop in human inspectors after four hours)
- Classification, trend analysis, and root cause identification
- 100% inspection capabilities replacing sampling approaches
- Continuous improvement through machine learning
- Predictive analytics powers:
- Forecasting potential failure points by analyzing hundreds of variables simultaneously
- Identifying complex interactions between multiple factors
- Detecting subtle anomalies that traditional statistical methods miss
- Simulating thousands of usage scenarios without physical testing
- Continuous learning from real-world performance data
- Natural language processing transforms customer feedback through:
- Analysis of millions of reviews, support tickets, and social media mentions
- Sophisticated sentiment analysis beyond simple positive/negative classification
- Topic clustering to organize unstructured feedback without predefined categories
- Multilingual capabilities identifying cross-cultural patterns
- Real-time alerts for development teams when issues emerge
- Machine learning revolutionizes user experience testing via:
- Pattern recognition identifying obstacles users don’t articulate
- Clustering algorithms revealing distinct user behavior patterns
- Anomaly detection finding unusual interaction patterns
- Predictive capabilities forecasting long-term user adoption
- Democratization of UX insights across organizations
- Automated test optimization delivers:
- Comprehensive test case generation identifying edge cases human engineers miss
- Efficiency gains with 30-40% fewer test cases while maintaining coverage
- Adaptive testing that dynamically adjusts based on results
- Test prioritization focusing resources for maximum effectiveness
- Continuous improvement in testing effectiveness
- Sensory analysis quantifies subjective perceptions through:
- Computer vision algorithms assessing visual attributes
- Audio analysis evaluating sound qualities objectively
- Taste and smell prediction from compositional data
- Cross-modal correlation revealing interactions between different senses
- Personalization for distinct consumer preference segments
- Future developments include edge AI, quantum computing applications, generative AI suggesting design modifications, human-AI collaboration models, and frameworks ensuring ethical implementation
- Vantagem competitiva – Organizations embracing AI methods gain compound benefits in development speed, insight discovery, and institutional knowledge that continuously improves testing effectiveness
What Makes SIS International a Top AI-Powered Testing Partner?
As AI transforms product testing capabilities, selecting a research partner with technological sophistication and methodological expertise has become crucial for companies seeking competitive advantage. At SIS International Research, we’ve invested heavily in developing proprietary AI testing capabilities that deliver unprecedented insights while maintaining the human touch essential for strategic interpretation.
Here’s why forward-thinking organizations choose SIS International for AI-enhanced product testing:
- Global AI Implementation Network – Our presence across major global markets allows us to implement AI testing protocols with local adaptation, ensuring algorithms account for regional variations in consumer behavior and product usage patterns while maintaining consistent methodological standards worldwide.
- 40+ Years of Testing Evolution – Our four decades of experience provide the historical testing data that powers our AI algorithms, creating models trained on decades of product performance correlations across industries. This rich historical foundation enables more accurate predictive capabilities than systems built on limited datasets.
- Advanced Participant Recruitment Systems – Our proprietary global databases leverage AI-matching algorithms to identify ideal test participants based on thousands of variables rather than basic demographics alone, ensuring testing panels truly represent your target users in ways traditional recruitment cannot match.
- Multilingual Natural Language Processing – With in-country staff supporting 33+ languages, our NLP systems analyze consumer feedback with native-language nuance detection, identifying sentiment patterns and product issues that cross-language analysis frequently misses or misinterprets.
- Proprietary Global Data Analytics Platform – Our custom-built analytics environment integrates multiple AI methodologies (machine learning, computer vision, NLP) into a unified insights ecosystem, revealing multidimensional patterns in testing data that single-algorithm approaches cannot detect.
- Human-AI Collaborative Framework – Unlike fully automated testing systems, our approach pairs AI analytical power with human strategic interpretation, creating a symbiotic relationship where algorithms surface patterns for expert analysis rather than delivering black-box conclusions.
- Continuous Algorithm Refinement Process – We systematically validate AI predictions against actual market performance, creating a continuous improvement cycle that has demonstrably increased predictive accuracy year-over-year across all major product categories we test.
FAQs: AI Methods for Product Testing
How does SIS ensure AI testing systems don’t miss critical issues human testers might catch?
We use hybrid testing approaches combining AI and human expertise rather than relying solely on either. Our AI systems excel at processing vast amounts of data, identifying patterns, and maintaining consistency, while human experts contribute creativity, context understanding, and judgment in ambiguous situations. We’ve found that structured complementary testing—where AI and human testers focus on their respective strengths—delivers superior results to either approach alone. Additionally, our validation protocols test the AI itself against known issues to verify detection capabilities before deployment. This complementary approach creates a safety net ensuring critical issues don’t fall through cracks while maximizing efficiency.
What’s the typical return on investment timeframe for implementing AI testing methods?
Most clients see ROI within 4-8 months, though this varies by industry and application. The fastest returns typically come from computer vision inspection systems, where quality improvements and labor efficiencies create immediate impact. Predictive analytics applications usually show returns within 6-12 months as prevented field issues translate to warranty savings. The highest long-term ROI comes from integrated systems that both improve quality and accelerate development cycles. One consumer electronics manufacturer calculated a 380% first-year return primarily through development acceleration and reduced warranty costs. The key to rapid ROI is starting with focused applications addressing specific pain points rather than attempting complete testing transformation simultaneously.
Do AI testing methods require massive historical data sets to be effective?
While more data generally improves AI performance, effective implementation doesn’t always require massive historical datasets. We use several approaches for situations with limited historical data: transfer learning applies knowledge from related products or industries; synthetic data generation creates training examples for rare conditions; active learning maximizes information from limited samples; and hybrid models combine physical understanding with available data. Using these techniques, one medical device manufacturer successfully implemented AI testing with just 14 months of limited historical data. The systems then improve as they collect more data during operation, creating a virtuous cycle of increasing accuracy.
How does SIS address potential bias in AI testing systems?
Addressing bias is critical for testing integrity. Our methodology includes several safeguards: diverse training data representing the full product usage spectrum; explicit bias testing by analyzing performance across different product variations; regular algorithmic audits by teams separate from the development group; continuous monitoring for performance drift; and maintaining human oversight of critical decisions. We’ve developed specialized tools to identify potential bias in test results and implement correction mechanisms when necessary. One consumer goods client discovered through our bias analysis that their AI system was less sensitive to certain defect types on darker-colored products—an issue we corrected through targeted algorithm refinement and training data augmentation.
Can AI testing methods be implemented for small production runs or highly customized products?
Absolutely—contrary to common perception, AI testing can be particularly valuable for small runs and customized products. We use few-shot learning techniques that require minimal examples to identify critical patterns; parameterized models that understand product variations rather than requiring examples of every variant; and adaptive learning systems that quickly adjust to new products. One luxury goods manufacturer successfully implemented AI quality inspection for runs as small as 500 units, achieving higher detection rates than manual inspection despite the limited volume. The key is designing systems that understand the underlying principles of quality rather than simply memorizing specific products.
How does SIS integrate AI testing with existing quality management systems?
Integration with existing systems is essential for maximizing value. Our implementation approach includes several key elements: standardized data interfaces connecting AI systems with existing quality databases; workflow integration ensuring insights reach the right teams at the right time; staged implementation that gradually increases AI responsibility; comprehensive staff training focusing on collaboration with AI tools; and clear protocols defining when human judgment overrides AI recommendations. We recently helped a manufacturing client integrate AI testing with their existing quality management system, resulting in a seamless workflow where the AI enhances rather than disrupts established processes. The goal is always augmentation of existing quality systems rather than replacement.
What privacy and security measures protect proprietary data used in AI testing systems?
Data protection is paramount when implementing AI testing systems. Our comprehensive approach includes: on-premises deployment options keeping sensitive data within client firewalls; advanced encryption for any data transferred or stored; strict access controls limiting exposure to sensitive information; data minimization principles collecting only necessary information; robust anonymization techniques for user data; and contractual frameworks clarifying data ownership and usage limitations. For particularly sensitive applications, we’ve implemented federated learning approaches where the AI models are trained locally without centralizing data. These measures create a secure environment for leveraging AI’s capabilities without compromising intellectual property or user privacy.
Localização de nossas instalações em Nova York
11 E 22nd Street, andar 2, Nova York, NY 10010 T: +1(212) 505-6805
Sobre SIS Internacional
SIS Internacional oferece pesquisa quantitativa, qualitativa e estratégica. Fornecemos dados, ferramentas, estratégias, relatórios e insights para a tomada de decisões. Também realizamos entrevistas, pesquisas, grupos focais e outros métodos e abordagens de Pesquisa de Mercado. Entre em contato conosco para o seu próximo projeto de pesquisa de mercado.