Decision Trees Analysis in Market Research
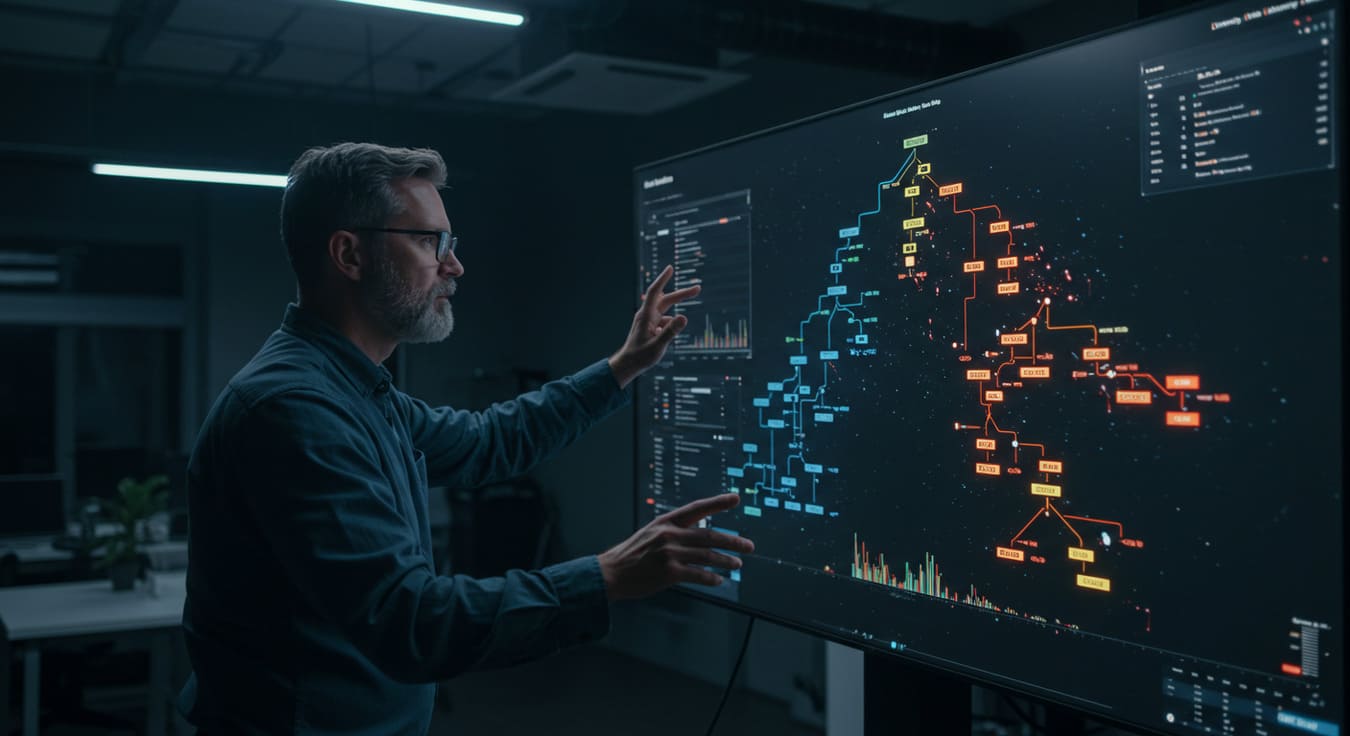
Decision trees analysis in market research is the most underutilized weapon in modern business strategy—and it’s costing companies millions in missed opportunities every day.
Have you ever stared at a mountain of market research data and thought, “What the hell am I supposed to do with all this?” Most researchers have. Many times. This is where decision trees analysis in market research becomes your lifeline. Not just another fancy statistical method—it’s the difference between data paralysis and strategic clarity.
Table of Contents
✅ Listen to this PODCAST EPISODE here:
What Are Decision Trees Analysis in Market Research?
Most businesses think customer decisions work in a rational, weighted-average kind of way—like some magical algorithm where people carefully consider all factors simultaneously.
Humans don’t make decisions that way. We eliminate options in sequences. We take mental shortcuts. We follow decision rules like “if this, then that” without even realizing it. Decision trees analysis in market research captures this messy, sequential reality in a way traditional methods can’t touch.
Unlike linear regression or factor analysis, decision trees don’t make assumptions about relationships between variables. They let the data speak for itself, revealing natural groupings and sequential decision criteria that might be completely counterintuitive.
How Decision Trees Transform Market Research Data
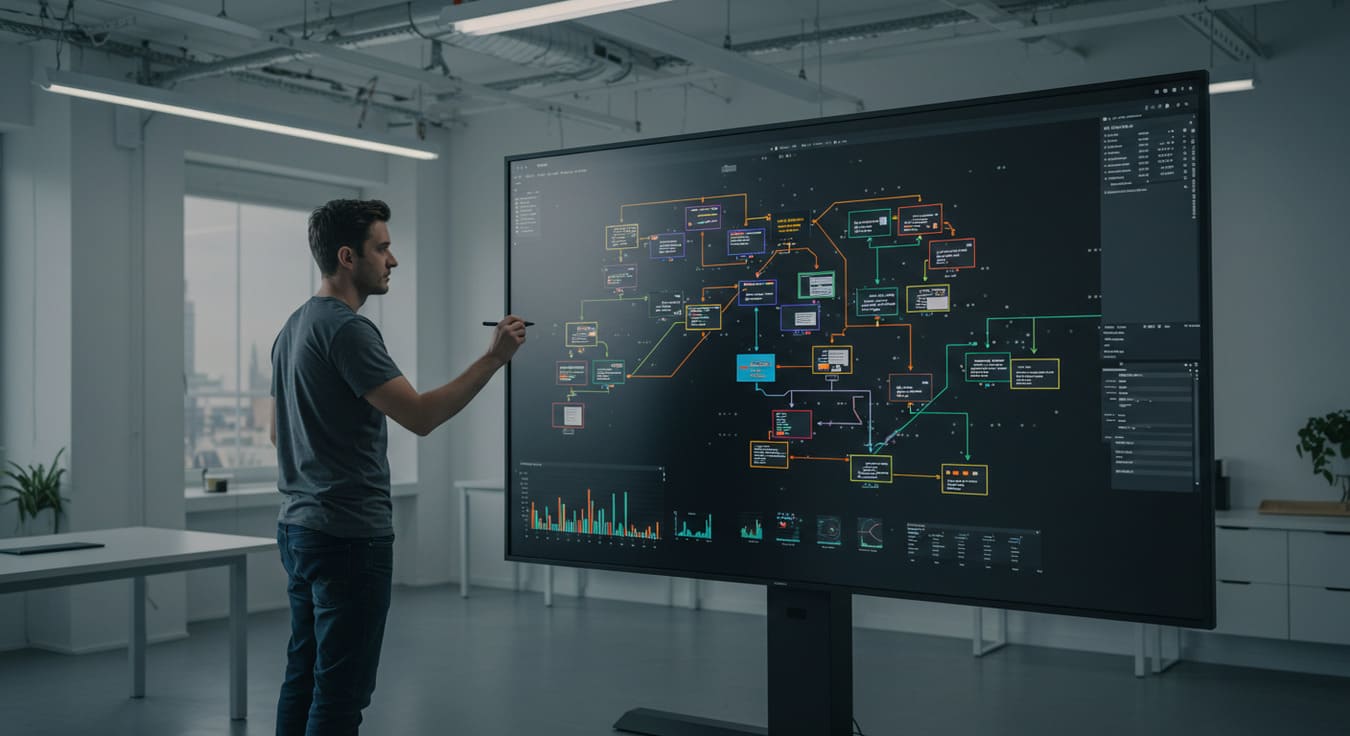
If your research isn’t answering these questions, you’re just collecting trivia—interesting, perhaps, but ultimately useless for driving growth.
Most research methodologies give you disconnected puzzle pieces. Decision trees analysis in market research shows you how those pieces fit together into actual customer journeys.
Here’s the unvarnished truth after four decades in this business: the difference between mediocre research and game-changing research isn’t the data—it’s how you extract meaning from it. And nothing extracts actionable meaning like decision trees.
Let me show you a case study. A healthcare client came to us last year with a genuinely puzzling situation. Their patient satisfaction scores were above industry average, yet their retention rates were plummeting. Traditional analytics showed no obvious correlations. They had mountains of survey data but zero useful insights.
When we applied decision trees analysis in market research to their patient journey data, the picture transformed completely. What emerged was a classic “poison branch” scenario: for 58% of patients, a single negative interaction with the billing department after treatment completely negated all positive clinical experiences.
The shock on the client’s face was priceless. “You mean the actual healthcare quality barely matters if billing screws up?”
For this particular decision pathway—yes, exactly.
So, the power of decision trees analysis in market research comes from its ability to show these conditional relationships. It’s not “what factors matter” but “what factors matter WHEN and FOR WHOM.”
Key Benefits of Decision Trees Analysis in Market Research
The most immediate benefit? Clarity amid complexity. Decision trees cut through the noise with brutal efficiency. Another game-changing benefit is identifying “tipping point” thresholds in customer decision processes. Traditional research might tell you price sensitivity exists on a spectrum. Decision trees reveal the exact price points where customer behavior changes dramatically.
Perhaps most valuable is how decision trees analysis in market research democratizes insights across organizations. Operations teams that normally glaze over during research presentations become deeply engaged when shown decision trees relevant to their function. The visual, intuitive nature of the methodology makes complex customer behavior accessible to everyone from the C-suite to frontline staff.
There’s also the benefit of predictive power. By mapping the decision architecture of different customer segments, you can forecast with remarkable accuracy how these segments will respond to new offerings or market changes.
Finally, there’s the competitive advantage that comes from addressing what actually drives decisions rather than what competitors are focusing on. As noted in industry publications, companies using advanced methodologies like decision trees gain significant market share advantages by solving for actual customer decision criteria while competitors chase perceived needs.
Implementing Decision Trees in Your Research Strategy
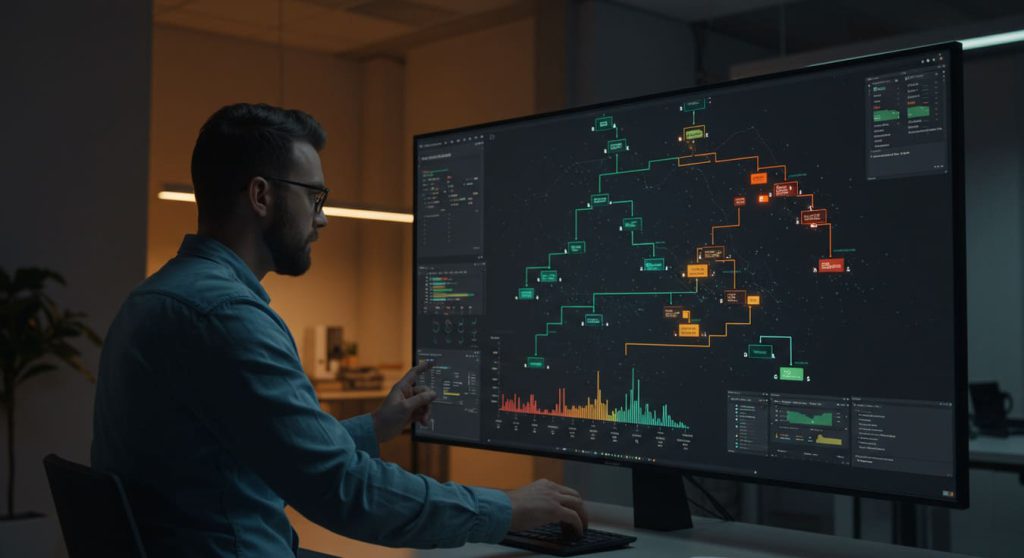
Let’s cut through the academic nonsense and talk about how you actually implement decision trees analysis in market research in the real world.
First, accept that your first attempt will be wrong. You’ll never see a perfect first-draft decision tree. The beauty of this approach is that it’s iterative—each version gets you closer to the truth of how customers actually make decisions.
Start with a clear outcome variable—the actual behavior you’re trying to understand or influence. Not attitudes, not preferences, not hypothetical intent. Actual. Behavior. For an e-commerce client, that might be purchase completion.
Next, gather data that captures both the outcome and potential predictor variables. This can come from multiple sources:
- Transaction data showing actual customer choices
- Digital journey tracking revealing navigation patterns
- Survey data connecting attitudes to reported behaviors
- Customer service interactions highlighting pain points
- Sales process documentation showing objection patterns
The magic happens in how you structure this investigation. Traditional approaches ask, “What factors matter?” Decision trees analysis in market research asks, “What factors determine the next step in the decision process?” It’s a subtle but crucial difference.
Implementation typically spans 4-12 weeks depending on data availability and complexity. The most successful programs follow this sequence:
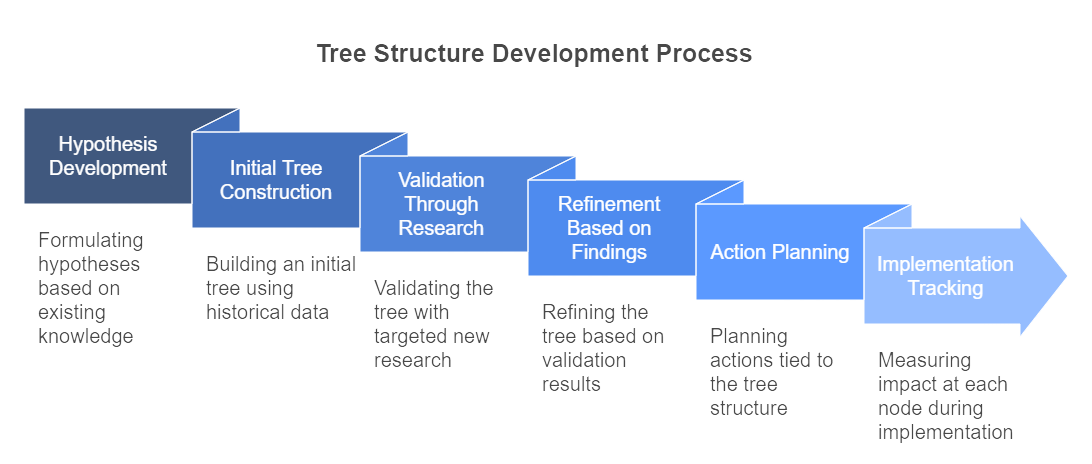
- Hypothesis development based on existing knowledge
- Initial tree construction using historical data
- Validation through targeted new research
- Refinement based on validation findings
- Action planning tied directly to tree structure
- Implementation tracking measuring impact at each node
The common pitfall? Analysis paralysis. many companies get so enamored with the statistical elegance of their decision trees that they forget the whole point is to drive action. A good decision tree should directly inform:
- Which product features to prioritize
- How to sequence marketing messages
- Where to focus CX improvement efforts
- Which customer segments need different approaches
Decision Trees Analysis in Market Research: Common Challenges and How to Overcome Them
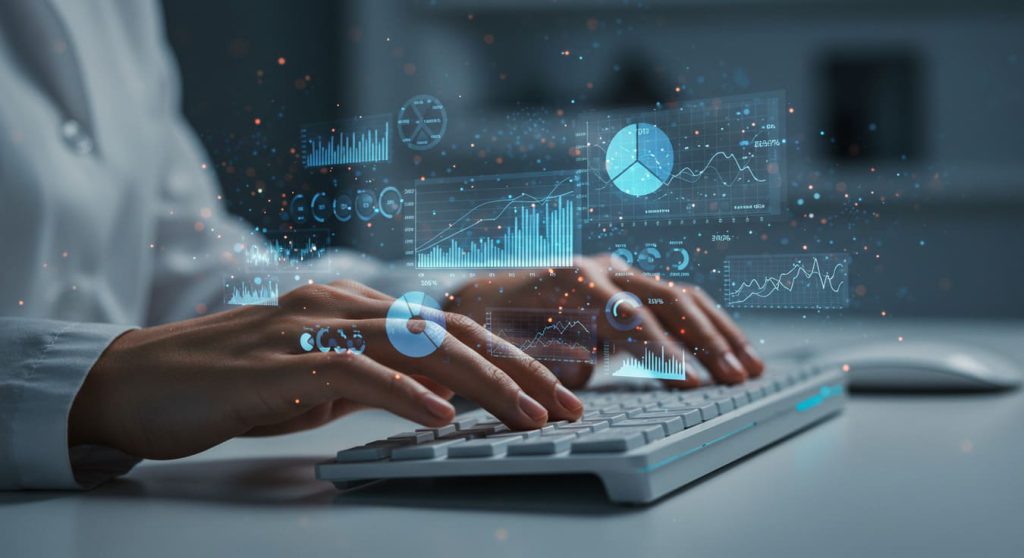
Data fragmentation is often the first and biggest hurdle. Your customer information likely lives in multiple systems that don’t talk to each other—CRM data here, transaction data there, survey responses somewhere else entirely. Before you can build meaningful decision trees, you need a consolidated view of the customer journey.
Organizational resistance presents another significant challenge. Decision trees analysis in market research has a nasty habit of invalidating pet projects and cherished assumptions.
Interpretation challenges often emerge even after successful implementation. You will see flawlessly constructed decision trees gathering dust because business teams couldn’t connect the analysis to concrete actions. The solution? Create “decision activation guides” that explicitly map tree nodes to specific business initiatives, eliminating the interpretation gap.
Perhaps the most insidious challenge is confirmation bias. Humans have a remarkable ability to see what they expect to see, even in objective data. To combat this, implement blind validation protocols where analysis teams don’t know the company’s existing hypotheses, and business teams evaluate multiple alternative tree structures without knowing which emerged naturally from the data.
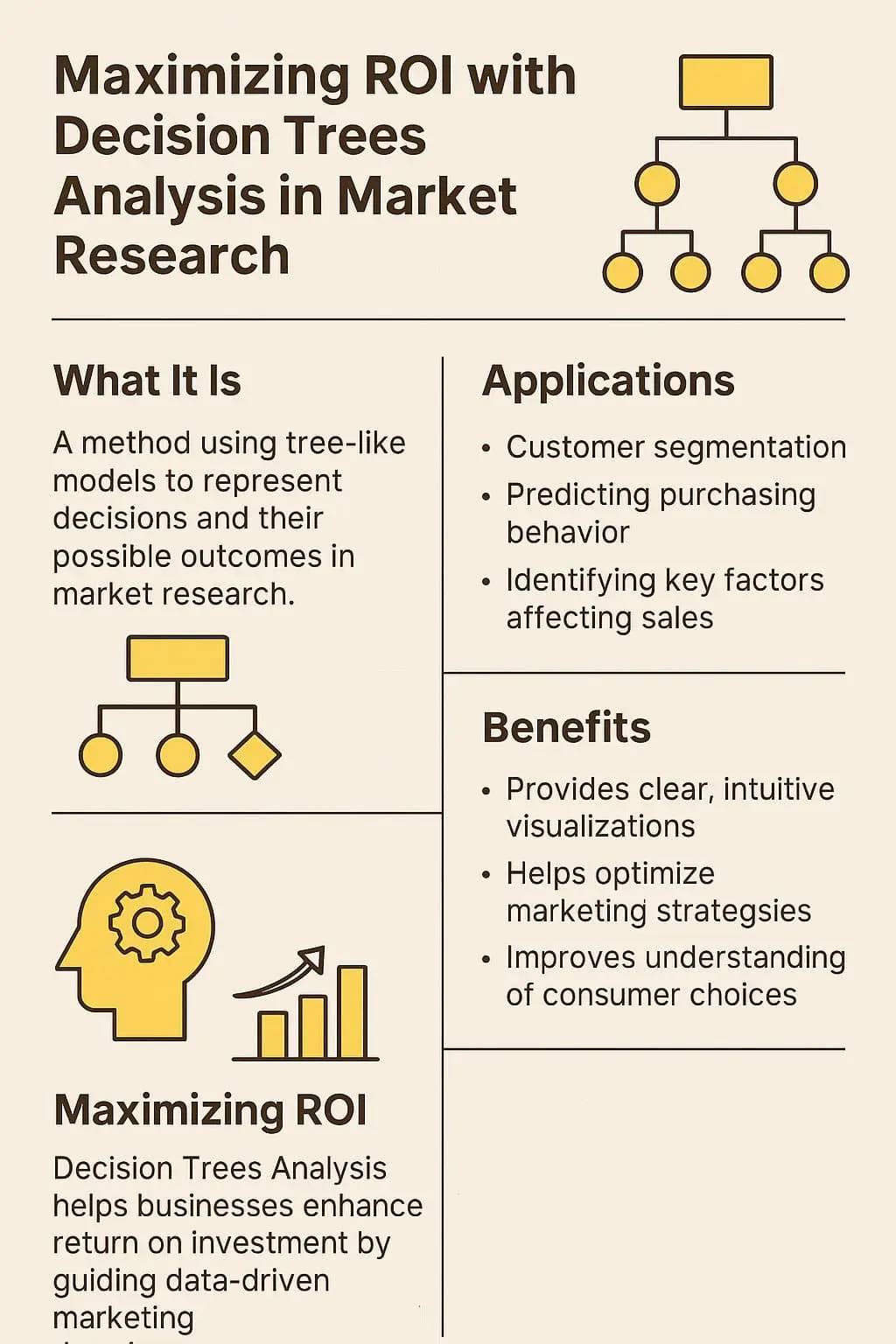
Key Takeaways: Decision Trees Analysis in Market Research
✅ Decision trees analysis in market research reveals not just what factors influence customer choices, but the actual sequence and structure of the decision process—information that traditional research methods completely miss
✅ This approach transforms mountains of disconnected data into clear decision maps showing exactly where to focus improvement efforts for maximum business impact
✅ Implementing decision trees requires both technical expertise and business context—it’s as much art as science to identify the right variables and validation approaches
✅ Advanced applications including real-time trees, ensemble models, and AI-enhanced analysis are dramatically expanding the predictive power and practical application of this methodology
✅ Common implementation challenges include data fragmentation, organizational resistance, and interpretation difficulties—all solvable with the right approach
✅ Future trends point toward dynamic, contextual decision trees that continuously update as market conditions and customer behaviors evolve
What Makes SIS International a Top Decision Trees Analysis Provider?
When you’re selecting a partner for decision trees analysis in market research, experience isn’t just a nice-to-have—it’s everything. After four decades pioneering these methodologies across industries and continents, here’s what actually sets us apart:
✔ GLOBAL REACH: Most market research firms claim “global capabilities” while actually subcontracting everything outside their home markets. Our teams have boots-on-ground presence in 120+ countries, capturing decision patterns across wildly different cultural contexts.
✔ 40+ YEARS OF EXPERIENCE: We’re not just applying textbook methodologies—we’ve helped write the damn textbooks. Since implementing our first decision tree models in the early 1980s, we’ve refined these approaches through thousands of projects.
✔ GLOBAL DATA BASES FOR RECRUITMENT: Decision trees are only as good as the data feeding them. Our proprietary respondent databases give us unrivaled access to specialized decision-makers others can’t reach.
✔ IN-COUNTRY STAFF WITH OVER 33 LANGUAGES: Decision-making is inherently cultural and linguistic. Nuances get lost in translation. Our native researchers capture decision criteria that would be completely missed by translated research.
✔ GLOBAL DATA ANALYTICS: Our dedicated analytics teams specialize in every variant of decision tree methodology, from classic CHAID and CART approaches to cutting-edge machine learning implementations.
✔ AFFORDABLE RESEARCH: Our global scale and methodological efficiency allow us to deliver sophisticated decision tree analysis at price points significantly below typical management consulting rates. We’ve structured our approach to deliver maximum insight value without the bloated timelines and teams that drive up costs elsewhere.
✔ CUSTOMIZED APPROACH: We don’t believe in one-size-fits-all methodologies. Each decision tree project is tailored to your specific business questions, data environment, and implementation context.
FAQs: Decision Trees Analysis in Market Research
What exactly is decision trees analysis in market research?
Forget the textbook definition. Decision trees analysis in market research is a way to decode how people actually make choices—not how they say they make choices, not how you wish they made choices, but how they really make them in the messy real world. It creates a branching map showing the exact sequence of considerations that lead to specific outcomes.
Think of it as an X-ray of your customer’s decision process, revealing which factors they consider first, which come later, and which knock products out of consideration entirely. Unlike traditional research that might tell you “quality matters,” decision trees show you precisely when quality matters in the decision journey and for which customer segments it’s a make-or-break factor versus just a nice-to-have.
How does decision trees analysis differ from other market research methods?
Most research methods give you disconnected data points. Surveys tell you what people say matters. Focus groups give you rationalized explanations for past behavior. Conjoint analysis shows feature preferences in artificial scenarios. All useful, but all incomplete. Decision trees analysis in market research is fundamentally different because it maps the actual structure of decision-making.
Traditional methods might tell you price sensitivity exists—decision trees show you the exact point in the journey where price becomes the determining factor. Regression analysis identifies correlations—decision trees reveal causal sequences. It’s the difference between having puzzle pieces scattered across the table versus seeing the complete picture of how those pieces fit together into actual customer journeys.
What types of business questions are best answered using decision trees?
Decision trees shine when you need to understand “why” and “how” rather than just “what.” They’re ideal for questions like: Where exactly are we losing customers in the purchase funnel? Which product features actually drive purchase decisions versus which are just nice-to-have? How do different customer segments follow completely different decision paths? Which marketing messages should we lead with based on actual decision sequences? What are the knockout criteria that eliminate products from consideration regardless of other strengths?
Essentially, any business question that involves understanding sequential decision processes or prioritizing improvement efforts will benefit enormously from this approach. It’s particularly valuable when you have limited resources and need to focus investments where they’ll have maximum impact on customer behavior.
How much data do we need to create reliable decision trees?
Here’s the good news—decision trees can actually work with smaller samples than many other quantitative methods. While traditional statistical approaches might require thousands of responses for reliable segmentation, decision trees can produce actionable insights with as few as 200-300 respondents if the decision structure is relatively straightforward. That said, more complex scenarios with numerous variables or low-incidence outcomes will require larger samples.
Can decision trees help with pricing strategy?
Abso-fucking-lutely. Decision trees are among the most powerful tools for sophisticated pricing strategy because they reveal not just price sensitivity but the exact role price plays in the decision structure. Traditional approaches might tell you customers are price-sensitive. Decision trees show you whether price is the first thing they consider (making you essentially a commodity) or whether it only matters after other criteria are satisfied (allowing premium positioning for the right features).
How long does it take to implement decision trees analysis?
I won’t sugarcoat this—doing it right takes time. For projects using existing data, expect 3-6 weeks from initiation to actionable insights. Projects requiring new data collection typically span 6-12 weeks depending on complexity and respondent accessibility. Implementation of the resulting insights varies dramatically based on what you discover and your organization’s agility.
That said, one advantage of decision trees is how quickly organizations can act on the findings. Because the results directly map to decision points, there’s minimal “translation” required between insight and action. The most successful implementations follow a phased approach: start with a focused application to demonstrate value, then expand methodically.
How do we measure the ROI of decision trees analysis?
Typical ROI manifests in several ways: Increased conversion rates when you focus improvements on actual decision drivers rather than assumed ones (we typically see 15-40% improvements); reduced marketing waste when messages align with actual decision sequences (20-30% efficiency gains are common); accelerated product development when features are prioritized based on decision impact rather than general preferences (reducing time-to-market by as much as 60%); and pricing optimization by understanding exactly where and how price influences decisions (typically yielding 5-15% margin improvements).
For precise measurement, we recommend establishing baseline KPIs for key nodes in your decision tree before implementing changes, then tracking improvements at each stage rather than just measuring final outcomes.
สถานที่ตั้งโรงงานของเราในนิวยอร์ก
11 E 22nd Street, ชั้น 2, นิวยอร์ก, NY 10010 T: +1(212) 505-6805
เกี่ยวกับ เอสไอเอส อินเตอร์เนชั่นแนล
เอสไอเอส อินเตอร์เนชั่นแนล เสนอการวิจัยเชิงปริมาณ เชิงคุณภาพ และเชิงกลยุทธ์ เราให้ข้อมูล เครื่องมือ กลยุทธ์ รายงาน และข้อมูลเชิงลึกเพื่อการตัดสินใจ นอกจากนี้เรายังดำเนินการสัมภาษณ์ การสำรวจ การสนทนากลุ่ม และวิธีการและแนวทางการวิจัยตลาดอื่นๆ ติดต่อเรา สำหรับโครงการวิจัยการตลาดครั้งต่อไปของคุณ